Home > Medical Research Archives > Issue 149 > Emerging Value-Based Radiology in the Era of Artificial Intelligence
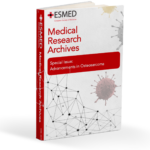
Published in the Medical Research Archives
May 2023 Issue
Emerging Value-Based Radiology in the Era of Artificial Intelligence
Published on May 26, 2023
DOI
Abstract
Radiology has a long history of adopting state-of-the-art digital technology to provide better diagnostic services and facilitate advances in image-based therapeutics throughout the healthcare system. The radiology community has been developing diagnostic artificial intelligence (AI) tools over the past 20 years, long before AI became fashionable in the public press. Currently, there are approximately four hundred Food and Drug Administration approved imaging AI products. However, the clinical adoption of these products in radiology has been relatively dismal, indicating that the current technology-push model needs to evolve into the demand-pull model. We will review the current state of AI use in radiology from the perspective of clinical adoption and explore the ways in which AI products will become an ensemble of critically important tools to help radiology transition from volume-based service to value-based healthcare. This transition will create new demands for AI technologies. We contrast the current “technology-push” model with a “demand-pull” model that will aligns technology with user priorities.
We summarize the lessons learned from AI experience over the past twenty years, mainly working with computer-aided detection for breast cancers and lung cancers. The radiology community calls for AI tools that can do more than detection with increasing attention toward higher workflow efficiency and higher productivity of radiologists. Major radiological societies of North America and Europe promulgated the emerging concept of value-based radiology service, an integral part of overall value-based healthcare. The transition to value-based radiology will happen and that higher value will come from the effective use of AI throughout the radiology workflow.
The value-based radiology will need to work with a full range of machine learning tools, including supervised, unsupervised, and reinforcement learning, as well as natural language processing and large language models (e.g., chatbots). The engineering community is rapidly developing many concepts and sophisticated software tools for data orchestration, AI orchestration, and automation orchestration. Current radiology operation has been supported by PACS, a monolithic IT infrastructure of past generations. This system will need to migrate to an intelligence management system to support the new workflow needed for high value radiology.
Author info
Author Area
Have an article to submit?
Submission Guidelines
Submit a manuscript
Become a member