Home > Medical Research Archives > Issue 149 > Evaluation of Control Measures Against Influenza Epidemic by Multiagent Simulation
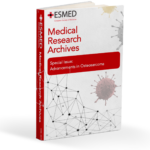
Published in the Medical Research Archives
Jul 2023 Issue
Evaluation of Control Measures Against Influenza Epidemic by Multiagent Simulation
Published on Jul 11, 2023
DOI
Abstract
Background: At the campus of Japan Coast Academy in January 2017, 37 of 150 students developed influenza (situation A). Besides, 18 of 56 students at the training ship and 13 of 109 students at the campus of the academy developed influenza in January 2019 at same time (situation B and C). The rare data of the three situations including behavioral history data of students, daily clinical data of influenza-positive students and social contact data of students were collected and directly analyzed. Then, using a proposed spatio-temporal Susceptible – Exposed – Pre-infectious – Infectious – Recovered (SEPIR) model, the incubation period and the infectivity rate from asymptomatic people were calculated. And multiagent simulation (MAS) of influenza epidemic has been performed using spatio-temporal SEPIR model and has been validated.
Aim: Effective non-pharmaceutical interventions to prevent influenza epidemic is described.
Methods: The epidemic is defined using ratio of daily patients in the community. Then, situation A and B are classified as epidemics, but situation C is not. Using the validated MAS, three measures of infection control (preventive measure such as wearing mask, school closure when 20 % of patients are discovered and that when first patients are discovered) against influenza in the two epidemic situations A and B are evaluated. The criteria for evaluation are the frequency of epidemic and the ratio of total patients.
Results: The frequency of epidemic by the school closures as infection control measure is 100 %, whereas, those by the preventive measure are low (4.5 % and 22.9 %) in two epidemic situations. The ratio of total patients by the school closures are above 80 % and those by the preventive measure are low (7.6 % and 23.0 %) in two epidemic situations.
Conclusions: MAS suggests that the preventive measure such as wearing masks, washing hands and better ventilating rooms is useful. Then, the preventive measure is recommended after winter vacation or three-day weekend in January not school closure.
Author info
Introduction
For coronavirus disease 2019 (COVID-19), infectious disease countermeasures are performed and we experience the effect. Basic preventive measures, such as wearing masks, washing hands and better ventilating rooms is effective, as well. Of course, vaccination is effective against infectious disease. Katow points out the importance of having a record as a lesson from the history of the new infectious disease and the records of past infectious diseases must be taken advantage for the countermeasures1. Japan Coast Guard Academy (JCGA) is 4-year undergraduate institution that educates and trains students of coast guard service as officers in Japan. JCGA is a residential college and all students live in the dormitories. The enrolled students were between the ages of 18 and 20. At JCGA in January 2017 and 2019, many students developed influenza2,3. No teachers or staffs who had some contact with the students developed similar symptoms at the time. Though, temporary school closures were not implemented, the epidemic contained by the basic preventive measure.
After the influenza epidemics, data which scattered in various departments including behavioral history data of students, clinical data of influenza-positive students and social contact data of student were organized and recorded2,3. Almost all data of students could be gotten due to small number of students, which are very rare because it is very difficult to obtain. Using proposed spatio-temporal Susceptible – Exposed – Pre-infectious – Infectious – Recovered (SEPIR) model and focusing on the super-spreading phenomenon, the data were analyzed by not statically but directly. Then, it is showed that the infectivity rate before the day that the first patients are discovered (hereinafter referred to as D-day) is high. Then, the infectivity rate becomes low due to the basic preventive measures3.
As for the method, a multiagent simulation (MAS) is performed, which is one of the research field of artificial intelligence. It can study of the evolution of the epidemic under different conditions. In this study, agent represents real student and agents move to their classrooms or dormitories and contact with other students, then MAS reproduce the spread of infection and validated4. In this study, to quantitatively evaluate the effect of different intervention measures using the validated MAS, three non-pharmaceutical interventions measures against influenza epidemic are evaluated.
Background
Seasonal influenza is a preventable infectious disease, mainly involving respiratory symptoms. Caused by the influenza virus which is moderately infectious, influenza is predominantly spread via droplets and contacts, or indirectly via respiratory secretions on hands, tissues, etc. Aerosol transmission can also play a part in spread of the virus5. The numbers of deaths due to influenza in many countries are gradually increasing in the 21st century, though they have been decreasing since 19606. Whereas, in the 1980s and after 2020, the number of patients of influenza is very small in Japan. Regarding the former, it is showed that mass immunization at school suppressed outbreaks and nationwide in the 1980s in Japan7. From 1962 to 1987, most Japanese schoolchildren were vaccinated against influenza. For more than a decade, vaccination was mandatory, but the laws were relaxed in 1987 and repealed in 1994. The effect of vaccination of schoolchildren is confirmed in other country8. After the 1990s, deaths among the elderly people due to influenza increased and influenza-associated encephalopathy of the infant become problems in Japan9.
There is ample prior evidence that children play a primary role in influenza transmission, as well10-12. High rates of transmission of children13 are due to more exposure potential and less prior immunity in these children compared with adults14. In other words, it is expected that reducing school epidemic can suppress a nationwide influenza epidemic. The large number of patients of influenza relate to increasing deaths among the elderly people due to influenza and influenza-associated encephalopathy of the infant. Regarding the latter, after 2020, Takeuchi et al15 show that there was an association between preventive infection control measures, such as high mask use, social distance and stringency of measures, with low influenza detection against COVID-19 after 2020. And they show that seasonal influenza is controllable through effective preventive measures.
Figure 1 shows the annual number of influenza cases per influenza sentinel sites by gray line. There are about 5,000 influenza sentinel sites in Japan16. The number of influenza cases increases in the 21st century. Figure 1 also show the number of temporary school closures, such as classes, grades and schools of the fourth week in the 52- week by influenza-like diseases in Japan, by black line, the thousand and right axis17. The number of influenza reports per influenza sentinel site of the fourth week is the highest in the 52-week17. In 2009, temporary school closures of high school were added to the report, which is shown by black dashed line. Temporary school closure increases in the 21st century. Influenza appears to be a biennial epidemic. The establisher of a school may temporarily close all or part of the school when necessary for the prevention of infectious diseases as temporary school closures in Japan. In many cases, classes, grades and schools are closed when the absentee rate reaches 20 %18. Figure 1 shows that the number of influenza patients at schools is increasing in the 21 centuries and influenza appears to be a biennial epidemic in Japan, as well.
Figure 1 Annual number of cases of influenza per sentinel site and the number of temporary school closures of the fourth week in the 52-week by influenza-like diseases in Japan. Excludes infectious diseases such as Avian influenza and novel influenza.
Related works
In an influenza epidemic report at a British boarding school in 1978, symptoms, graph of daily positive cases and daily new recovery cases were recorded19. Of 763 students returned from all over England and some Europe and the Far East for the new school term starting on January 10th. There was one student returning from abroad developed a fever from January 15th to 18th. Then, within two weeks, 512 students developed Flu. Keeling et al used a mathematical model of infectious diseases, Susceptible – Infected – Recovered (SIR) model20 to obtain the mean transmission rate and the average infectious period and showed that the basic reproduction number is classified as human influenza21. The basic reproduction number is the average number of secondary infections produced by one infected person in a healthy population21. Giesecke points out that the mathematical model of infection is not realistic with respect to the hypothesis that any member of a population has an equal chance of contacting any other member and the hypothesis that there is only one type of contact. He said that there is little data on contact patterns in particular22. Originally, the transmission rate is consisting of the contact rates and transmission probability21.
In a report of a novel influenza epidemic at the United States Air Force Academy (USAFA) in 2009, symptoms, graphs of daily new positive cases and the number of positive cases per squadron were recored23. Of 1376 cadets arrived on June 25th to participate six weeks training prior to the start of first academic year. There was one cadet suspected to be positive on June 26th and 134 were confirmed positive within one month. There, attack rate per squadron is calculated. The attack rate is used in epidemiology and is the percentage of those who developed the disease out of those exposed to the factor22. The attack rate used in epidemiology depends on how precisely exposure and disease onset were ascertained22 and time units for the attack rate are not usually provided24.
In a report of H1N1 influenza epidemic at a Chinese university in 2009, there were 586 cases of H1N1 influenza-like illness in the outbreak, in which 226 cases were identified to be H1N1 influenza illness25. Among them, 105 cases lived in No.7 dormitory building. 1506 students in the dormitory came from 31 Chinese provinces. The distribution of 105 cases in No.7 dormitory building is described. Assignments of dorms and classrooms of students, the time schedule, population proportion among provinces and the invention policy at the time are described. Then, they simulated H1N1 influenza epidemic and compare the results with data to verify the models, including spatial models, population distribution, weighted social networks, contact patterns and students behaviors. In the three reports, the history of clinical data for each student are not recorded, which are expected. This may be because of the large number of students. Then, routes of infection or transmission rates cannot be calculated due to the lack of enough personal contact data. In the real world, we often only have access to information about a subset of those exposed to personnel, a subset of those who develop the disease22. In such situations, when calculating risk, we must assess how accurate and reliable the risk is, then it requires statistic22.
In the reports of influenza epidemic at JCGA in 2017 and 2019, the history of clinical data for each student were recorded2,3 because of small academy. It is likely that students who were infected off-campus brought in influenza virus to the JCGA on January (three-day weekend or winter vacation) in 2017 and 2019, when influenza is prevalent nationwide. At the JCGA, influenza-positive patients are quarantined to the infirmary, then transmission does not occur from those who are positive. However, influenza epidemic occurred because it can be transmitted from those who have the ability to transmit it even if they have not yet developed the disease26. And it is hard to be given the effect of border measures for influenza because of asymptomatic people.
The data which scattered in various departments and including behavioral history data of students, clinical data of positive people and social contact data of students were collected, organized and recorded. By a proposed spatio-temporal SEPIR model of influenza2,3, the data were analyzed. Then, it is showed that the influenza epidemic at JCGA was an on-campus outbreak, with most infections occurring in classrooms. The incubation period and the infectivity rate from asymptomatic people is calculated2. Then, it is reported that simple averages are not valid for calculating infectivity rates and that detailed data analysis is necessary.
Focusing on the super-spreading phenomenon, it is showed that the infectivity rate before D-day is high and many students were infected3. After D-day, a few students were infected3. Students were directed to start basic infectious disease control measures such as wearing masks, washing hands and better ventilating rooms, amongst other measures, when the first patients are discovered. There, temporary school closures werent implemented. The basic control measures seem to take effect after about one day and the infectivity rate become lower and the epidemic was contained2,3. Of course, vaccination is effective in infectious disease outbreaks7,8. It is showed that with the Artificial Intelligence (AI) technology, machine learning methods (including deep learning) and MAS are among the most relevant from a methodological standpoint. Machine learning methods have been applied to detect patterns and analyze trends and risks from public health surveillance data. MAS allow for the study of the evolution of the epidemic under different conditions and can be used to quantitatively evaluate the effect of different interventions and control measures27.
Numerous MAS25,28-33 of epidemic have been reported. Almost MAS are based on SIR20, Susceptible – Exposed – Infectious – Recovered (SEIR)21 or SEIR-like model and simulates stochastically the spread of a contagion in large and social contact networks25,29-33. Almost MAS use transmission rates based on attack rates. The attack rate used in epidemiology depends on how precisely exposure and disease onset were ascertained22 and time units for the attack rate are not usually provided24 as Rothman has noted. And the social contact networks are based on census data, which is big data. In epidemiology, there is not enough data on contact patterns for epidemics as Giesecke points out22. Data that is easily accessible and insightful is called small data34. Big data focuses on finding correlations, whereas small data focuses on finding causal relationships and is beginning to be used these days. However, small data is difficult to collect.
As for the influenza epidemic at JCGA in 2017 and 2019, by examining infections by a pre- infectious people using small data, MAS of
influenza epidemic in closed spaces is performed and basic simulation is validated. In this study, effective non-pharmaceutical interventions measures against influenza epidemic is examined using MAS with spatio-temporal SEPIR model. In the next section, the SEPIR model for a seasonal influenza is presented. And epidemic is defined and epidemic situation is described in Section 5 and simulation setting, MAS and the parameters is shown in Section 6. Control measures of influenza and simulation results is shown in Section 7. Then, discussion is described in Section 8 and finally, conclusion of this study is described.
Proposed SEPIR Model
Traditional epidemic mathematical model is SIR model20 and the state transition diagram of an individual is shown in Figure 2(a). Keeling et al21 deal with incubation period and propose mathematical SEIR model which many childhood infectious diseases (such as measles, rubella, or chickenpox) follow. The state transition diagram of an individual is shown in Figure 2(b). In those models, only an infected I individual can infect a susceptible S individual.
The pre-infectious state P, that is asymptomatic was introduced into the SEIR model and proposed the discrete mathematical SEPIR model for influenza2. The incubation period is divided into two periods, the exposed period and the infectious period, with neither having any symptoms. The former was set as the exposed state E and the latter as the pre-infectious state P. The state transition diagram is shown in Figure 2(c).
Figure 2 The state transition diagram of an infected individual: the circles show the state of individual. S: Susceptible state, E: Exposed state, P: Pre-infectious state, I: Infectious state and R: Recovered state. The black circle mean that they can affect others.
An individual of I or P can infect a S individual. The probability of contact between individuals of susceptible S and infectious I is determined by their respective numbers. Considering a mean infectivity rate β, an individual of S moves to exposed E, which is shown in Eq (1) 20. The probability of contact between individuals of S and P is also determined by their respective numbers. By introducing infectivity rate α, an individual of S moves to E as given in Eq (1)2. By introducing transmission rate σ, an individual of E moves to P as given in Eq (2)2. By introducing transmission rate τ, an individual of P moves to I as given in Eq (3)2. By introducing recovery rate γ which is the inverse of the infectious I period, this leads to a far more straightforward equation as shown in Eq (4) 20. Here, S(t), E(t), P(t), I(t) and R(t) is the number of individuals of S, E, P, I and R, t representing day.
5 Epidemic and situations
5.1D- day
By retrospective investigation of pre-infectious students, some students are super-spreading influenza until the day that first patients are discovered, which is defined D-day. Students were directed to start basic preventive measure, such as wearing masks, washing hands and better ventilating rooms, amongst other measures, when the first patients are discovered at JCGA. After D- day, the measures seem to take effect after about one day and the infectivity rate become lower. Then, after D-day, a few students were infected3.
5.2 Epidemic
According to the American Public Health Association in USA, an epidemic is defined as a situation in which the number of disease outbreaks in a given community exceeds a standard35. Ministry of Health, Labour and Welfare in Japan has established a threshold based on past patient outbreaks, as well. Here, I define the epidemic level by the ratio in population36. The epidemic level is 6.7 % of the community population for reference to previous studies3 and an epidemic is defined as a situation in which the daily number of new positive cases exceeds the level after D-day.
5.3 Situation A2
During January, 2017, an epidemic of influenza occurred at JCGA2. JCGA is a residential college. At that time, there were 150 undergraduate students, consisting of 56 freshmen, 47 sophomores, 44 juniors and just 3 seniors due to the others being on away for on the ship training. After a three-day holiday from January 7th to 9th, students returned to their dormitories and resumed to take classes. On Friday January 13th, two students, a freshman and a sophomore, showed symptoms of influenza and D-day is January 13th. 37 students, that is 20 freshmen, 13 sophomores and 4 juniors, showed symptoms in the next two weeks. Here, January 14th, 15th and 22nd are day off. Figure 3(a) is epidemic data in situation A. Gray bar refers to the number of new patients, is new Infectious I students, which is calculated using calculated incubation period. Black dashed line refers to the number of patients, that is Infectious I students. The epidemic level (6.7 %) of JCGA is indicated by gray dashed line. As shown in Figure 3(a), there is a day when the epidemic level is exceeded, that is January 15th, and the epidemic can be confirmed.
5.4 Situation B3
During January, 2019, an epidemic of influenza also occurred on a JCGA training ship3. After winter vacation until January 3rd, students returned to the academy and started embarkation training. On Thursday January 10th, one student showed signs of influenza, namely, D-day is January 10th. Within two weeks there were 18 cases out of 56 freshmen. Here, January 5th, 6th, 12nd, 13rd, 14th, 19th and 20th are day off. Figure 3(b) is epidemic data in situation B. There are two days, that is January 13rd and 14th, when the epidemic level is exceeded and the epidemic can be confirmed.
5.5 Situation C3
During January, 2019, a separate influenza epidemic occurred at JCGA3. At that time, there were 109 undergraduate students excluding freshmen (54 sophomores, 51 juniors and just 4 seniors) as the others were conducting on board training. During January 2019 the freshmen (in situation B) had no contacts with other students and were trained by different staff. After the winter vacation until January 3rd, students returned to the dormitories and resumed to take classes. On Wednesday January 9th, six students exhibited influenza and D-day is January 9th. 13 students, that is 11 sophomores and 2 juniors, showed symptoms within two weeks. Here, January 5th, 6th, 12nd,13rd, 14th and 20th are day off. Figure 3(c) is epidemic data in situation C. There is no day when the epidemic level is exceeded after D- day and the epidemic didnt occur.
(a) Situation A (150 students).
(b) Situation B (56 students).
(c) Situation C (109 students).
Figure 3 Daily new patients and patients: data and simulation results.
SD: Standard deviation
Method
6.1 Multiagent Simulation (MAS)
An agent is a physical entity that we wish to view in terms of its perceptions and actions37. And agent decides about what action to perform based on the history of the system, containing agents and the environment38. The society of agents in the compute or in silico is observed as laboratories, which is MAS39. In MAS, behaviors of society emerge from the interaction of agents in the environments under rules with bounded rationality of information and calculation. The aim is to discover fundamental local mechanisms generating the behavior. MAS involve three basic ingredients: agents, an environment or space, and rules. Each agent has internal state and behavioral rules. Some states are fixed and others depend on other agents to interact and the environment. Agents can also change the behavioral rule as agents move around and interact. The environment, the medium under with agents interact, can be a more abstract structure, such as a communication network. Environment is a medium separate from the agents, on which the agents operate and with which they interact. There are rules of behavior for the agents and for environment.
6.2 Simulation environment
I used artisoc40, which is a MAS platform whose intuitive controllability allows ideas to be rapidly incorporated into models. Results of execute simulations can be obtained quickly by going through just three broad steps. With regard to the complicated configuration of simulation models and the output and display of simulation results, artisoc basically allows settings to be made easily using the GUI, permitting the model designer to focus on constructing the original model. Used computer is DELL Mobile Precision 5560 (Intel Core i7-11850H).
6.3 Settings
6.3.1 Daily ScHEDULE and timetable
An influenza epidemic using MAS, where each agent represents a student was simulated. Each agent follows the daily schedule as shown in Table
1. Each day, the students have a sleeping time of 8 h in their assigned dormitories. On weekdays, the students have up to four classes of 90 minutes in the classroom. All classes are compulsory. The timetable in situation A and C are shown Table 7 in reference 2 and Table 10 in reference 3. It is assumed that all students had the same practice as for timetable in situation B, due to usage restrictions.
Table 1 Simulated schedule for the students.
6.3.2 Social contact data of students
The dormitories and classrooms are considered as closed spaces with a corresponding risk of infection. The assignment of dormitories which students live in and the classrooms which students take classes is used as social contact data. Those in situation A is shown Table 5 in reference 2. Those in situation B and C are shown Table 6 and Table 9 in reference 3. In situation B, it is assumed that all students had the same practice and students are live in separate areas for men and women instead of living cabin number because it could not be obtained.
6.3.3 Seeds
Seeds who brought in influenza virus in the JCGA campus or the training ship before D-day were used4. Students 1 and 2 were exposed on January 9th and become E on January 10, students 3-7 were exposed on January 10th and students17 exposed on January 11th, 2017 in situation A. Three of them are fist-year students and four of them are second-year students in the seed of situation A. Students 1 and 2 were exposed on January 6 and become exposed on January 7th, 2019 in situation B. They are first-year students. Students 1-6 were exposed on January 5 and become exposed on January 6th, and students 7-9 were exposed on January 6, 2019 in situation C. They are all 2-year students. Students exposed after D-day were excluded from the seeds.
6.3.4 Duration of Simulation, Number of trials and other parameters
The duration between January 10th and 26th, 2017 for situation A and that of January 6th and 23rd, 2019 for situation B and C were simulated. 10,000 trials for each situation were performed. Situation B is regarded as a single school years classroom, whereas simulation A or C requires dealing with multiple school years. According to the former study, it is regarded that students are infected in one classroom with the largest number of students in a day4. The simulation is performed using a spatio- temporal SEPIR model with parameters as shown in Table 1, 2 and 3, which are calculated by epidemic data2,3. Students were directed to start basic preventive measures such as wearing masks, washing hands and better ventilating rooms, amongst other measures, when the first patients are discovered. After D-day, the measures seem to take effect after about one day and the infectivity rates become lower. In situation C, not many students are infected at last, as the infectivity rates is low before D-day. Infectivity rate β is set as 0, as influenza-positive patients are quarantined to the in-firmary at JCGA.
Table 2 Infectivity rate α and recovery rate γ2,3
Table 3 Other parameters2
6.3.5 Criteria
The frequency of epidemic and the ratio of total patients in average are used. The epidemic is defined as a situation in which the daily number of new positive cases exceeds the level after D-day, where the epidemic level is 6.7 % of the community population. And the frequency of epidemic is the ratio of trials that epidemic occurred in the duration of simulation out of 10,000 trials. The ratio of total patients is the ratio of average of total patients in the duration of simulation out of 10,000 trials.
6.3.6 Aim and scope
The aim of this study is to seek effective non- pharmaceutical interventions to prevent influenza epidemic. As for scope of this study, the social contact network is defined by social contact in closed spaces, such as classrooms and dormitories. The physical distance between students are not considered, as the data were collected. As for the infectivity rate of students are set as uniformity. This study is based on real data and useful for prediction of influenza epidemic in small society such as school or community. But, it is difficult to simulate in big society such as prefecture or country.
6.4 Validated simulation
Table 4 shows the simulation results. The frequency of epidemic and the total patients in average are shown. The simulation result in situation A shows that epidemic occur in all trials and 31.7 % of students are infected in average in the simulation duration. The simulation result in situation B shows that epidemic occur in almost all trials and 68.9 % of students are infected in average in the simulation duration. The simulation result in situation C shows that epidemic rarely occurs and 10.6 % of students are infected in average in the simulation duration.
Table 4 Frequency of epidemic and the ratio of total patients in validated simulation.
Before Figure 3 shows simulation results, as well. The white bar refers to the average of new I students and the error bar refers to the twice standard deviation (SD) of new I students. Almost all data are within the twice SD and the simulation is validated, especially in situation B and C. Black line refers to the average number of “I” students and dotted line refers to the twice SD. Almost all data are within the twice SD of simulation, especially in situation B and C. Then, the simulation is validated4. In situation A, there is a little delay, I will analyze it in the future work.
6.5 Without measures
Simulation results without measures is shown in Table 5. The infectivity rate α in classrooms or dormitories are set as 0.041 independent of days. In situation A, epidemic occur in all trials and 90.0 % of students are infected in average in the simulation duration. In situation B, epidemic occur in all trials and 98.6 % of students are infected in average in the simulation duration. In situation C, epidemic occur in all trials and 80.5 % of students are infected in average in the simulation duration. In situation C, the number of total patients is lower than that of situation A. This is because there are smaller number of students in the classrooms or dormitories than that of situation A and B. This refers the effect of small rooms. As a whole, the results show that almost all students are infected within two weeks if people dont take measure.
Figure 4 shows simulation results without measures. The white bar refers to the average number of new I students and the error bar refers to the twice SD. Black line refers to the average number of I students and dotted line refers to the twice SD of simulation. The results show that the number of new patients exceed many times in situation A and B, that is, epidemic occur many times if people dont take measure.
Table 5 Frequency of epidemic and the ratio of total patients without measures.
(c) Situation C (109 students).
Figure 4 Simulation results without measures. SD: Standard deviation
7 Evaluation of measures
6.4 Measures
Following three measures are adopted for evaluation as shown in Table 6 for reducing the number of patients. Simulation in situation A and B are performed where epidemic occurred as shown in section 5.
• Preventive measure: Measure 1
After the winter vacation, basic preventive measures such as wearing masks, washing hands and better ventilating rooms are implemented. The infectivity rate α in classrooms or dormitories is given by Table 6, which is same as that after D-day of Table 2. And it is similar to situation C, as well where epidemic didnt occur. This is defined as preventive measure of epidemic. If no one have onset of symptoms for several days, the preventive measure will stop.
• Temporary school closure
Temporary school closure of the year or the academy is implemented once after the patients are discovered as shown in Table 6. There is no rule about the period and it depends on the establisher. Generally, the period of temporary school closure is between two and five days in Japan41. In this study, the period of the closure is set as five days. Here, two types of temporary school closure as for the timing is adopted to the simulation. The infectivity rate α in classrooms or dormitories is given by Table 6, it is same as that before D-day of Table 2.
─ Measure 2
In Japan, when about 20 % of patients are discovered, a temporary school closure is carried out. Then, the timing of the temporary school closure is set when the 20 % of patients are discovered in the year or the academy as shown in Table 6.
─ Measure 3
In the beginning of the outbreak of COVID-19 in Japan, if students of positive COVID-19 are discovered, a temporary school closure is carried out. Then, the timing of the temporary school closure is set when the first patient is discovered as shown in Table 6.
Table 6. Infectivity rate α and temporary school closure.
6.5 Simulation Results
6.5.1 frequency of epidemic and total patients
The simulation result in situation A is shown in Table 7. The frequency of epidemic and ratio of total patients in average are shown. By measure 1, the new patients exceed 447 trials out of 10,000 trials. The ratio of total number of patients in average is 7.6 % of students within two weeks. By measure 2, epidemic occur in all trials and the ratio of total number of patients in average is 80.7 % of students in the simulation duration. By measure 3, epidemic occur in all trials and the ratio of total number of patients in average is 86.7 % of students in the simulation duration.
Table 7 Frequency of epidemic and the ratio of total patients in situation A.
The simulation result in situation B is shown in Table 8. By measure 1, the new patients exceed 2,294 trials of 10,000 trials. The ratio of total number of patients in average is 23.0 % of students within two weeks. By measure 2, epidemic occur in all trials and the ratio of total patients in average is 96.1 % of students in the simulation duration. By measure 3, epidemic occur in all trials and the ratio of total patients in average is 95.0 % of students.
Table 8 Frequency of epidemic and the ratio of total patients in situation B.
6.5.2 New patients and patients
Figure 5 shows simulation results by measures in situation A. The dashed bar refers to the average of new I students, that is new patients, and the error bar refers to the twice SD. Black bold line refers to the average number of I students, that is patients, and dotted line refers to the twice SD. The epidemic level (6.7 %) of students is indicated by gray dashed line. By measure 1 as shown in Figure 5(a), it is confirmed the measure is effective after a three- day holiday in January. The number of new patients doesn’t exceed the epidemic level and the result is similar to that of situation C as shown in Figure 3(c). By measure 2 as shown in Figure 5(b), the number of new patients exceed the epidemic level many times, that is, epidemic occur many times. The 20% of students is indicated by black solid line. On January 17th, the infected students in average exceeds 20 % of students, then temporary school closure is implemented from January 18th to 22nd in average. Before the temporally school closure, the number of infected students exceeds 20 % of students two times (on January 16th and 17th), namely epidemic occur two times. During the temporary school closure, epidemic occur two times (on January 20th and 22th). After finished the temporary school closure, the number of new infected students doesn’t exceed the epidemic level and epidemic don’t occur. The result is similar to the results of without measure as shown in Figure 4(a).
By measure 3, the number of new patients exceed many times, that is, epidemic occur many times as shown in Figure 5(c). On January 13th, first student onset. Then, temporary school closure is implemented from January 14th to 18th. During the temporally school closure, epidemic occur two times (on January 16th and 17th). Though temporary school closure is finished, there are new infected students on January 19th. After finished the temporary school closure, epidemic occur three times (on January 20th, 22th and 23th). Temporary school closure may be implemented again after January 19th. The result is similar to the results of without measure as shown in Figure 4(a).
(c) Measure 3
Figure 5. simulation results with measure in situation A.SD: Standard deviation
(c) Measure 3
Figure 6. simulation results with measure in situation B. SD: Standard deviation.
Discussion
8.1 Infectivity rate
According to the definition of epidemic, situation A and B are classified as epidemic, but situation C is not. No other studies have been found on the infectivity rate from asympto-matic people of influenza, but there are some studies about that of symptomatic. Among them, some studies show that younger (under 20 years old) people have high infection rate11,12, in which people are classified into two categories, under 20 and over 20 years old.
Students’ data are not about ages at JCGA, but the age of students relates the school year. The seed who brought in influenza virus were first- or second-year students and many first- or second- year students developed in situation A. However, upperclassmen were infected at JCGA. If third-year students or fourth-year students borough influenza virus, something similar could happen. On the other hands, no teachers or staffs who had some contact with the students didn’t develop influenza at that time. It is considered that students are younger regardless of year and teachers or staffs are older. Based on the SEPIR model, younger peo-ple with high infectivity rate is easy to be infected. On the contrary, older people with low infectivity rate is difficult to be infected. Then, it is expected that reducing school epidemic can suppress a nationwide influenza epidemic.
In aforementioned influenza epidemic reports, the epidemic of influenza occurred after the new term. Then, for effective control measure, it is necessary focusing on the new term, which students gather from various places. At JCGA, that is early January. At JCGA, in January 2023, many students developed influenza though it is not classified ep- idemic. Except during the COVID-19 epidemic, influenza appears to be a biennial epidemic. This point is interesting and it seems to be contribution for control measure.
8.2 School closure
The simulation results in situation A is reliable than that of situation B, as the settings of classrooms and dormitories in situation B are provisional3. The reason is that real data during the ship training was not used. The setting of the dormitories is higher than the capacity, which is the capacity of each cabin is about four. Therefore, I will focus on situation A. By measure 2 and 3, simulation results show that temporary school closure doesnt stop epi-demic. The probability of epidemic is 100 % if influenza virus is brought in to the commu-nity. And the total number of patients is above 80 % of students.
The study suggests that school closures are not effective against the spread of COVID-1942. The another study, which is analysis of data from 37 countries on COVID-19 cumulative mortality during the first pandemic wave, shows that early application of mass gatherings bans and school closures in outbreak epicenters was associated with reduction in COVID-1943.The other study which reviewed 45 papers about the effects of school closure on influenza outbreaks as predicted by simulation studies suggests that school closure can be a useful control measure during an influenza pandemic, particularly for reducing peak demand on health services44. However, it is difficult to accurately quantify the likely benefits.
The ratio of patients in the peak is shown in Table 9 in this study. By measure 2 and 3 in sit-uation A, the ratios of patients in the peak are about 38%. The reducing ratio by measure 2 is 15.2 % regarding that of without measure, while temporally school closure doesn’t stop epi-demic, the role of reducing peak can be confirmed and the benefits can be calculated. The total patents are similar by measure 2 and 3 in situation A. This shows that the effect by temporary school closures, that is measure 2 and 3, in situation A are similar despite of timing of school closure.
Table 9 Patients in the peak in situation A.
The other study showed that the effect of school closure result from the timing and duration of school closure as well45. Then, for the benefit of reducing the peak, early school closure is necessary and for the duration of school closure, long duration is necessary. In Japan, duration of temporary school closure is between two and five days41. For mitigation an influenza epidemic, duration of the temporary school closure in several simulation is set as over 20 days46,47. These studies suggest the effectiveness of long duration of school closure, though these are mitigation strategies against novel influenza.
By measure 3 in simulation results, when school closure is finished that is January 19th, there are 30 pre-infectious students in average. This is corresponding to that of January 15th, when the number of patients is increasing because pre- infectious students can infect others in the dormitories during school closure. The study shows that the success of school closure is depend on other social contact45,48. School closings would not have effect on epidemic without reducing other social contact47. Then, school closure should be operated without additional contacts such as contact at dormitories.
8.3 Basic preventive measure
It is confirmed that the measure 1 is effective after the winter vacation or a three-day holiday in January. This is preventive measure of epidemic. The probability of epidemic by preventive measure (measure 1) is very small if influenza virus is brought in to the community. And the ratio of total patients and the ratio of patients in the peak are small as shown in Table 9, as well. The result is similar to that of situation C, as the setting of infectivity rate is similar. It shows that the preventive measure is practical control. Takeuchi et al15 shows that there was an association between preventive infection control measures, such as high mask use, social distance and stringency of measures, with low influenza detection against COVID-19 after 2020. And they show that seasonal influenza is controllable through effective preventive measures.
As for the period of preventive measure, it is supposed that about five days. As shown in former study, the incubation period is three days2. Students who were infected off-campus brought in influenza virus to the JCGA in January develop influenza four days after the students returned. In fact, students exist who develop influenza five days after returned JCGA, as some students who onset lately, endure or don’t notice the symptom exist, or there are errors in data. And some studies support the mixed strategies for mitigation an influenza epidemic45,47,48. Here, when applied to general schools, classrooms are equivalent to school and dormitories are equivalent to homes. Then, it is recommended that preventive measure not only school but also homes for five days after winter vacation on January.
As settings of measure 1, the infectivity rate in classrooms is 0.002 and one twenty of that before D-day (0.041) in the validated simulation. And the infectivity rate in dormitories is 0.013 and one third of that before D-day (0.041) in the validated simulation. That of dormitories after D- day is seven times higher than that of classroom3, as the measures become negligent in dormitories. That is, control measure only in classrooms is not enough. This supports the mixed strategies for mitigation an influenza epidemic45,47,48. Therefore, it is effective to take preventive measure, such as masks, ventilation and hand washing five days after winter vacation or three- day weekend on January. If first patients are discovered in the five days, the measure has to be continued. Otherwise, first patients are not discovered in the five days, the measure can be end. In this study, the records of infectious diseases of students are able to be taken advantage for the countermeasures1.
9 Conclusion
By examining infections by a pre-infectious people using epidemic data at JCGA, MAS of seasonal influenza in closed spaces has been performed and basic simulation has been vali-dated. In this study, using validated simulation, three measures against influenza are evaluated, which has been difficult to accurately quantify the likely benefits. Then, the basic preventive measure is the most effective, such as wearing masks, washing hands and better ventilating rooms and implemented for five days after winter vacation or three-day weekend. It is recommended that preventive measure not only school but also homes for five days. As future work, to get help from students and departments at JCGA, we plan to conduct a demonstration experiment.
References
1. Katow S. History of corona virus diseases and lessons from COVID-19 pandemic. Jpn. J. History Pharm. 2021; 56(1): 1-6. in Japanese.
2. Iwanaga S, Kawaguchi K. Analysis of epidemic of seasonal influenza closed space. Proc 22nd IES. 2018; 37-44.
3. Iwanaga S. Super-spreading is possible by the day the first patients are discovered in the community. Journal of Advances in Artificial Life Robotics. 2022; 3(1): 24-31.
4. Iwanaga S. A research on multi-agent simulation of seasonal influenza from pre- infectious people in closed spaces. Vietnam Journal of Computer Science. 2023. in press.
5. ECDC. Factsheet about seasonal influenza. https: //www.ecdc.europa.eu/en/seasonal- influenza/facts/factsheet. Apr 12, 2022.
6. OECD stat. Health Status (Mortality). https: //stats.oecd.org/Index.aspx?DatasetCode=HE ALTH_STAT. July 4, 2022.
7. Reichert TA, Sugaya N, Fedson DS et al. The Japanese experience with vaccinating schoolchildren against influenza. N Engl J Med. 2001; 344: 889-896.
8. Monto AS, Davenport FM, Napier JA, Francis T Jr. Effect of vaccination of a school-age population upon the course of an A2-Hong Kong influenza epidemic. Bull World Health Organ. 1969; 41(3): 537-542.
9. Taya K. Influenza vaccination rate. Infectious Agents Surveillance Report. 2002; 23(12): 311-313. in Japanese.
10. Brownstein JS, Kleinman KP, Mandl KD. Identifying pediatric age groups for influenza vaccination using a real-time regional surveillance system. Am J Epidemiol. 2005; 162(7): 686-93.
11. Sullivan KM, Monto AS, Longini IM Jr. Estimates of the US health impact of influenza. Am J Public Health. 1993 Dec; 83(12): 1712- 6.
12. Fox JP, Hall CE, Cooney MK, Foy HM. Influenzavirus infections in Seattle families, 1975-1979. I. Study design, methods and the occurrence of infections by time and age. Am J Epidemiol. 1982; 116(2): 212-227.
13. Longini IM Jr, Koopman JS, Haber M, et al. Statistical influence for infectious diseases: risk-specific household and community transmission parameters. Am J Epidemiol 1988; 128: 845—859
14. Longini IM Jr, Halloran ME. Strategy for distribution of influenza vaccine to high-risk groups and children. Am J Epidemiol. 2005 Feb 15; 161(4): 303-6.
15. Takeuchi H, Kawashima R. Disappearance and re-emergence of influenza during the COVID-19 pandemic: Association with Infection Control Measures. Viruses. 2023; 15(1): 223.
16. NIID. Annual surveillance data (Sentinel- reporting diseases). https: //www.niid.go.jp/niid/ja/ydata/11532- report-jb2021.html. Feb. 3, 2023. in Japanese.
17. MHLW. Influenza outbreak. https: //www.mhlw.go.jp/stf/seisakunitsuite/bunya/k enkou_iryou/kenkou/kekkaku- kansenshou01/houdou_00010.html. Mar. 31, 2023. in Japanese.
18. Eto T, Nakahara T. Handbook for school medical needs for physicians and teachers. Tokyo: Bunkodo; 2006. in Japanese.
19. Epidemiology. Influenza in a boarding school. BMJ. Mar. 4, 1978; 587.
20. Kermack WO, McKendrick AG. A contribution to the mathematical theory of epidemics. Proc. R. Soc. Lond. 1927; A115700–721.
21. Keeling MJ, Rohani P. Modeling infectious diseases in humans and animals. Princeton: Princeton University Press; 2008.
22. Giesecke J. Modern infectious disease epidemiology. Boca Raton. CRC Press; 2017.
23. Witkop CT, Duffy MR, Macias EA et al. Novel influenza A (H1N1) outbreak at the U.S. Air Force Academy- epidemiology and viral shedding duration, AJPM. 2010; 38(2): 121- 126.
24. Rothman KJ. Epidemiology: An Introduction (2nd edition). Oxford: Oxford University Press; 2012.
25. Duan W , Cao Z, Wang Y et al. An ACP Approach to public health emergency management: Using a campus outbreak of H1N1 influenza as a case study. IEEE Transactions on Systems, Man, and Cybernetics: Systems, 2013; 43(5): 1028-1041.
26. CDC. How influenza Spreads. https: //www.cdc.gov/flu/about/disease/spread.htm. Sep 20, 2022.
27. Daniel Z, Zhidong C, Daniel BN. Artificial intelligence - enabled public health surveillance - from local detection to global epidemic monitoring and control, Artificial Intelligence in Medicine. London: Academic Press; 2021, 437-453.
28. Parker J, Epstein JM. A distributed platform for global-scale agent-based models of disease transmission. ACM Trans on Modeling and Computer Simulation. 2011; 22(1): 2.
29. Cliff OM, Harding N, Piraveenan M et al. Investigating spatiotemporal dynamics and synchrony of influenza epidemics in Australia: an agent-based modelling approach. Simulation Modelling Practice and Theory. 2008; 87: 412-431.
30. Barrett CL, Bisset KR, Eubank SG et al. EpiSimdemics: An efficient algorithm for simulating the spread of infectious disease over large realistic social networks. Proc of SC 08. 2008.
31. Bisset K, Chen J, Feng X et al. Epifast: A fast algorithm for large scale realistic epidemic simulations on distributed memory systems. Proc ICS 09. 2009; 430-439.
32. Chao D, Halloran M, Obenchain V et al. FluTE, a publicly available stochastic influenza epidemic simulation model. PLoS Comput Biol. 2019; 6(1): e1000656.
33. Nagai H, Kurahashi S. Measures to prevent and control the spread of novel coronavirus disease (COVID-19) infection in tourism locations, SICE Journal of Control, Measurement, and System Integration. 2022; 15(2), 1-12.
34. Bonde A. Defining small data, https://smalldatagroup.com/2013/10/18/defining -small-data. Oct 18, 2013.
35. Heymann DL(edit). Control of communicable diseases manual: An official report of the American public health association (21th). Washington DC, American Public Health Association; 2022.
36. MHLW. Influenza/ Influenza season and peak season (nationwide). https: //www.mhlw.go.jp/content/000620809.pdf. Mar 31, 2023. in Japanese.
37. Russell SJ. Subramanian D. Provably bounded-optimal agents. Journal of Artificial Intelligence Research. 1995; 2: 575-609.
38. Wooldridge M, An Introduction to multiagent systems, 2002. Wiley
39. Epstein JM, Axtell R. Growing artificial societies, Social science from the bottom Up. Washington DC, Brookings Institution Press & MIT Press; 1996
40. Kozo Keikaku Engineering Inc. Artisoc 4. https: //mas.kke.co.jp/en/artisoc4. Mar 31, 2023.
41. Nikkyo web. What you need to know in order not to panic even if a class closure occurs. https: //www.kyoiku-press.com/post-211110/. Jan 3, 2020. in Japanese.
42. Fukumoto K, McClean CT, Nakagawa K. No causal effect of school closures in Japan on the spread of COVID-19 in spring 2020. Nat Med. 2021; 27: 2111–2119.
43. Piovani D, Christodoulou MN, Hadjidemetriou A et al. Effect of early application of social distancing interventions on COVID-19 mortality over the first pandemic wave: An analysis of longitudinal data from 37 countries. J Infect. 2021; 82(1): 133-142.
44. Jackson C, Mangtani P, Hawker J et al. The Effects of School Closures on Influenza Outbreaks and Pandemics: Systematic Review of Simulation Studies. PLoS One. 2014; 9(5): e97297.
45. Milne GJ, Kelso JK, Kelly HA, Huband ST, McVernon J. A small community model for the transmission of infectious diseases: comparison of school closure as an intervention in individual-based models of an influenza pandemic. PLoS One. 2008; 3(12): e4005.
46. Ferguson N, Cummings D, Fraser C et al. Strategies for mitigating an influenza pandemic. Nature. 2006; 442: 448–452.
47. Germann T, Kadau K, Longini I, Macken C. Mitigation strategies for pandemic influenza in the United States. PNAS. 2006;103: 5935– 5940.
48. Haber J, Shay D, Davis X, Patel R, Jin X. Effectiveness of interventions to reduce contact rates during a simulated influenza pandemic. Emerg Infect Dis. 2007; 13: 581– 589.
Author Area
Have an article to submit?
Submission Guidelines
Submit a manuscript
Become a member