Home > Medical Research Archives > Issue 149 > Testing Physicians’ Attention Deficit/Hyperactivity Disorder (ADHD) Practices with vs. without Care Manager Support
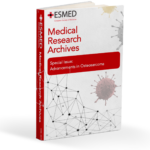
Published in the Medical Research Archives
Jul 2023 Issue
Testing Physicians’ Attention Deficit/Hyperactivity Disorder (ADHD) Practices with vs. without Care Manager Support
Published on Jul 11, 2023
DOI
Abstract
Objective: To understand the benefits of an intensive 6-months-long practice change intervention (with or without assistance of an embedded care manager) on primary care providers’ (PCPs’) adoption of evidence-based practices for diagnosing and managing Attention-Deficit/Hyperactivity Disorder (ADHD).
Methods: Following an intensive weekend training in primary care pediatric mental health service delivery, 47 PCPs were randomly assigned to 6 months of ongoing educational support via twice-monthly conference calls, either with or without additional assistance of a care manager. In addition to the impact of a care manager, basic science-derived predictors of behavior change were examined to explore impact on PCPs’ initial and subsequent intentions/decisions to adopt 11 specific changes in ADHD practices. Effects of practice obstacles on PCPs’ practice decisions, both initially and over 6 months, were also examined.
Results: PCPs’ initial and subsequent decisions to employ program-targeted ADHD evidence-based practices increased over time and were significantly predicted by underlying hypothesized predictors. Additional support of a care manager had minimal effects on PCPs’ initial and subsequent decisions to apply specific evidence-based ADHD practices. Of note, PCPs’ initial worries/perceptions concerning practice obstacles decreased significantly over time, likely due to twice-monthly support calls.
Conclusions: With intensive and sustained support, PCPs will adopt most evidence-based practices for ADHD diagnosis and treatment. Many initially anticipated obstacles dissipated over time. Additional support of care managers had little impact on PCPs’ longitudinal decisions to adhere to ADHD practice guidelines. Basic science predictors of behavior change are robust correlates of PCPs’ practice changes and should be more routinely applied to understand and improve training outcomes in multiple areas of health service delivery.
Author info
Introduction
Attention-Deficit/Hyperactivity Disorder (ADHD) is a prevalent mental health disorder among youth that is often managed in primary care settings.1 Although highly effective treatments have been identified and can be feasibly delivered in primary care settings,2 strong evidence indicates that assessment and treatment practices performed by primary care providers (PCPs) fall substantially short of optimal standards.2 Although multiple clinical practice guidelines have been published to improve the diagnosis and treatment of ADHD,3-6 PCPs struggle to implement guideline-based practices 7-11 for ADHD and other conditions. This should not be surprising since multiple research reviews have shown that neither published guidelines nor typical continuing medical education (CME) programs produce meaningful changes in health care providers’ practices. 12-18 In fact, this problem pervades not just PCP and mental health settings, but permeates all areas of medical practice.19
Underlying causes are many, 19 but it is noteworthy that most CME efforts and guideline dissemination efforts are limited by their general failure to address PCP-perceived practice barriers, such as time, support resources, and costs. Moreover, other relevant factors are rarely studied, such as PCPs’ personal beliefs about the guidelines (e.g., attitudes, beliefs, peer influences, self- efficacy, etc.) which can potentially interfere (or enhance) the adoption of new evidence-based practices. 12-18 Because most practice change studies have shown that new research findings and/or guidelines to PCPs generally do not change day-to-day clinical practices, we suggest that the practical problem of assisting PCPs to keep current with evidence-based guidelines should be reconceptualized from its status as an educational challenge -- to a behavior change problem. Some recent studies have taken the complexity of PCP behavior change into account, employing multi- pronged approaches—such as sustained interactions with physician experts (e.g., academic detailing), chart audits, patient-specific feedback, and hands-on practice in the targeted new behavior. When most or all these components are combined, the interventions do in fact lead to behavioral change, 18-22 but the resources to implement such approaches can be substantial – and totally infeasible if required for all healthcare provider practice changes needed across our medical systems. Moreover, although multiple studies of adult depression have effectively employed these more complex interventions, follow- up studies have yielded mixed results whether these approaches are universally cost-effective. 23-24
If as a field we wish to increase the effectiveness and affordability of our healthcare provider practice change interventions, it is essential that we conduct controlled studies of the single and additive effects of various intervention components. For example, can we conduct unpacking studies of the various components, taking some away, possibly adding others? Furthermore, to fully understand the benefits of such interventions, we must also carefully assess the dose of each component, just as is done in multiple medication/psychotherapy randomized clinical trials (RCTs), cancer trials, etc. By assessing each components dose/intensity and by also measuring the extent to which each component affects any known underlying processes and predictors of change (e.g., such as glucose or HbA1c levels in diabetes), such findings should allow us to make our PCP change studies more effective, efficient, and feasible.
If we do reconceptualize health care providers practice change as a behavior change problem, what do we know about the basic science underpinnings of why people change their behaviors – the processes/ingredients of change? Surprisingly, a great deal.
This field of research is nearly 50 years old, but has largely only focused on changing patients behaviors, e.g., encouraging diet, exercise, medication compliance, substance use desistance, and AIDS prevention (use of clean needles, condoms). 12,25 Several closely interlocking basic science theories have guided this body of behavior change research, such as Social Cognitive Theory, 26 the Theory of Reasoned Action, 27 the Theory of Planned Behavior, 28 the Health Beliefs Model, 29 the Transtheoretical Model (also known as Stages of Change), 30 and Rogers 31 Diffusion of Innovations Theory, and others. Regardless of these different names, theory developers and subsequent researchers generally agree on many of the key common elements predicting and affecting behavior change. 32-34 These elements were summarized and described at a National Institute of Mental Health conference attended by many of the leading theorists, 33-34 and many researchers who apply these consolidated basic science change models often refer to their combined common elements as the Unified Theory of Behavior (UTB) change, 34-37 also used in this report.
The relevance of UTB theory to PCP research is considerable: if malleable, underlying predictors of behavior change can be identified, measured, and targeted prior to and during PCP behavior change studies (or carefully designed CME programs), intervention impact might be improved over time by enhancing these factors. What are these malleable predictors? Predictors include PCPs attitudes and beliefs about the value of any proposed new practices (also called expected-values [EVs]) and include what the PCP perceives about the possible pros and cons of the new practice -- will it help or hurt patients outcomes? According to UTB, for a behavior change intervention to be effective, PCPs underlying beliefs/attitudes about the new practice must be understood by the intervention staff so that any incorrect or problematic beliefs/attitudes can be addressed, and that scientific information can be presented that leads to more positive attitudes. Delivering such science-based information is not simply a process of battering learners with many facts, but must instead be offered by appropriate messengers (i.e., credible experts) whom the learners like, trust, and are readily available to assist them throughout the behavior change process.
A second major UTB predictor of behavior change are the practice norms as perceived by the PCP. Will his/her colleagues approve of the PCP performing the new practice? Will his/her admired, local and/or national experts agree with this practice? Because perceived norms are well- established and malleable predictors of behavior change, many pharmaceutical representatives capitalize on these research findings by bringing key opinion leaders into office practices to speak with practitioners about the merits of a new medication.
A third key predictor of behavior change is self-efficacy, i.e., the extent to which the PCP believes that s/he can be effective and competent in performing the new practice. Obviously, if a PCP believes s/he cannot effectively perform a new practice, and if the teacher/trainer determines this during the intervention, the trainer must able and willing to provide training support to increase the PCPs new skills, and help him/her address any perceived obstacles. This is only possible if the PCP trusts the trainer sufficiently to divulge his/her skill needs and to request assistance. Literally hundreds of UTB and related theoretical studies 25 have shown that when these 3 predictive elements are enhanced, individuals will then be strongly motivated to perform the new practice, essentially deciding to change their behavior. Within UTB this internal decision is termed a behavioral intention (BI), and it can vary in strength, from weakly committed to strongly committed.
Care Managers:
Even after forming a strong BI, an individual may encounter obstacles to the new practice that slightly or greatly hinder performing it, such as time constraints, costs, patient reluctance to accept the PCPs recommendations, and even forgetting to do the new behavior during a busy day. The obvious nature of such obstacles has led health care planners to hire care managers (also sometimes called case managers, usually a nurse of social worker) to assist PCPs in time-consuming patient management tasks often needed for patients with chronic illnesses -- patient education/motivation, completion of additional tasks (e.g., completing forms and rating scales), facilitating specialty input/referrals (including mental health), encouraging treatment adherence, following up missed visits, etc. Not surprisingly, care managers are a central component of collaborative care approaches (and most other integrated behavioral health care models), as their inclusion has proven beneficial for treatment outcomes. 20-21
Figure 1: Unified Theory of Behavior
Predictors of Behavioral Intentions (BIs), as based on the Unified Theory of Behavior (UTB) Change: Expected- Values, Norms, and Self-Efficacy. Obstacles may hinder the translation of Behavioral Intentions into Behavior The Figure illustrates how the 3 UTB predictors of behavioral intentions (BIs) are linked to BIs, how BIs are linked to actual behaviors, and how potential obstacles can be conceptualized as affecting ultimate behavioral completion. Please note, more factors have been posited to affect BIs34 but this simplified model captures the most important elements.
The current report describes a longitudinal study that examines the relationship between PCPs self-rated UTB behavioral change predictors (expected-values, perceived norms, and self- efficacy) vis-à-vis PCPs BIs. Guided by one of the study co-authors (JJ), an expert in UTB theory application, below we present these measures as they were done 1) immediately following a 3-day- long, hands-on CME training, and 2) after 6-months of twice-monthly small group (8-10 PCPs) conference calls. During these twelve 1-hour calls (facilitated by 2 trainers), participants took turns presenting cases from their own practices. The training was specifically designed to augment the 3 predictors, in order to maximize their effects on PCPs BIs immediately after training and over the 6- months follow-up.
To test the potential additive effects of a specific practice support component (often requested by PCPs), immediately following the initial 3-day baseline training, all PCPs were randomly assigned to receive either: 1) 6 months of additional follow-up support provided by an ADHD care manager, vs. 2) no additional support (other than the twice-monthly small group conference calls). The care managers responsibilities are described in the Care Manager paragraph above. Goals and Hypotheses: In the current study, because UTB and related theories have rarely been applied to studies of PCP behavior change, we sought to determine the extent to which the 3 individual UTB predictors (individually and together) were linked to strong BIs, both at baseline and at 6 months follow-up. Second, we sought to determine the impact on PCPs by receiving (by random assignment) the support/assistance of a care manager, in terms of whether PCPs were more likely to change their ADHD practice behaviors. Finally, because we identified PCPs perceived obstacles to implementation of optimal ADHD practices at baseline, we examined whether these perceived obstacles changed over time, with or without the benefits of ADHD care management, and the extent to which perceived obstacles interfered with subsequent practice changes.
Methods
Participants
Participants included 47 PCPs associated within a network of primary care pediatric providers, one of multiple networks in the Community Care of North Carolina (CCNC) system caring for Medicaid recipients. The majority of providers were female (63.8%, n = 30) with an average age of 48.1 (SD = 9.6). Seventy percent of providers were trained as pediatricians, 11% trained in family medicine, and 19% trained as non-physicians (physician’s assistants, or nurse practitioners, all with prescribing privileges). Participants saw an average of 92 (SD = 48.2) pediatric patients per week. Thirty-nine of 47 participants (83%) provided data at both time points.
Procedures and Study Design
The study investigator (PSJ) and key leaders of the primary care network (SW, CH) recruited participants through emails, announcements, and phone calls. Participating PCPs attended a three-day training in Patient-centered Mental Health in Pediatric Primary Care (PPP), a program developed by a non-profit organization (REACH Institute) intended to enhance PCPs abilities to accurately assess and diagnose child mental health problems (see Love et al 38 for complete description of the training, and how UTB theory was used to guide all intervention components).
Following the 3-day training, PCPs were randomized to either (1) PCP phone call support only, consisting of 1) an additional 6 months of twice-monthly small group (10-12 PCPs) conference calls facilitated by 2 trainers, or 2) the same PCP support, augmented by the regular assistance of trained care managers (CMs) and office staff who were tasked to assist the PCPs in providing optimal care of their ADHD patients and families. Assigned CM duties included patient/family education about optimal ADHD diagnosis and treatment, following up with completion of parent and teacher ADHD questionnaires, initiating phone calls to families in the event of missed visits, ensuring medication adherence, and linking families when needed to mental health specialists. Please note, prior to their deployment, all CMs received one-day intensive training in optimal ADHD care, written materials for family education, and guidance in providing optimal support to their assigned PCPs. Following this 1-day initial CM training, they then received twice monthly one-hour group supervision and support for problem-solving and guidance, provided by the senior CM supervisor and a child psychiatrist study team member (PSJ).
Measures
Differences in expected-values, normative beliefs, and self-efficacy regarding ADHD assessment and treatment practices were assessed by participants self-completed surveys immediately following the 3-days’ training as well as at 6 and 12 months (only the first follow-up data are presented in this report). Respondents were paid a stipend for completing the packet of questionnaires at baseline and follow-up. All methods and questionnaires were IRB-approved. Questionnaires were developed to assess eleven BIs that were identified based on published ADHD guidelines, 3-6 following standard UTB methods. 27-28,33-34 Similarly, these questionnaires also assessed participants expected-values, normative beliefs, and self-efficacy pertaining to the 11 BIs. For example, one BI assessed is I will use parent rating scales to assess initial ADHD symptoms.
At baseline and at 6-months follow-up, BIs were measured on an 11-point scale (0 = do not intend to do/perform to 10 = definitely intend to do/perform) and refer to the extent in which providers intended to perform/use each of the recommended ADHD clinical practices. Similarly, Expected-Values were measured on an 11-point scale (0 = not at all likely to 10 = extremely likely) and addressed participants beliefs about how likely will positive consequences occur for your patients if the provider performed a given practice. Descriptive Norms were measured by prompting participants to provide their best guess as to the percentage of national experts and other providers who perform the recommended ADHD clinical practice. Other Norms were measured on an 11-point scale (-5 = strongly disapprove to 5 = strongly approve) referring to participants’ perception of how much their local practice colleagues would approve/disapprove the participant performing each of the 11 recommended ADHD clinical practices. Self-Efficacy was measured on an 11-point scale (0 = very hard to 10 = extremely easy) and refers to participants beliefs concerning how easy or difficulty it is to perform each of the 11 specific behaviors in their clinical practice.
The left column of Table 1 lists all 11 BIs.
A final additional questionnaire assessed participants perceptions concerning how often 10 specific obstacles might interfere with their implementing recommended ADHD clinical practices. This questionnaire, completed at baseline and 6-months follow-up, was also based on an 11-point scale (0 = never to 10 = always).
Data Analysis
First, to explore the theory-derived hypothesized effects of the 3 UTB predictor variables on PCPs behavioral intentions, zero order correlations between predictors and BIs were first calculated cross-sectionally and over time. Second, to test for possible changes over time in participant BIs from the initial 3-days training (referred to as T1) to six months follow-up (referred to as T2), mixed effects analyses examined the main effects of the 6-months’ training intervention (e.g., time) and CM assistance (yes/no), as well as their interactions (training x CM Y/N status). Third, to determine the overall explanatory power (and total explained variance) of the UTB model on changes in participants’ BIs over time, we first computed change scores for each UTB predictor variable by subtracting T1 from T2 scores and then entered them into multivariate linear regression analyses, along with participants CM status. Fourth, given the possibility that the longitudinal training and the presence or absence of CM assistance would affect PCPs’ perceptions of obstacles (increasing or reducing), we conducted mixed effects analyses to examine main effects of time (e.g., 6 months training), perceived obstacles, CM status, and their interactions.
Results
Analyses of the expected theoretical associations between the 3 UTB predictors and participants intentions/decisions (BIs) are shown in Table 1. 28 of the 44 zero-order were significant, with the most BI associations found for Expected- values (E-Vs) and self-efficacy. But as seen here, the actual correlations vary depending on the specific BI – as hypothesized and outlined in the UTB model. 33-37 For one behavior, practice norms may be quite important, while another behavior might occur outside of the awareness of colleagues, so E- Vs and self-efficacy may be more relevant. Also presented in Table 1 are the Time 1 means for all BIs and the 4 predictor variables. Of note, some BIs were quite high, but others were more moderate, suggesting more uncertainty and/or more variability among providers as to whether they could do the new practice at the end of the 3 days.
To further examine the power/relevance of the UTB model in this healthcare setting, Table 2 presents the zero order correlations of Time 2 BIs vis-à-vis both the Time 1 and Time 2 predictor variables. As one might expect, a greater number (n=19) of significant correlations were found for the T2 cross-sectional correlations between BIs and UTB predictors. Yet T1 UTB variables still yielded multiple (n=11) significant T2 BIs correlations, suggesting some BI stability.
Table 1: Time 1 UTBa Variable Means, & Zero Order Correlations between Baseline BIs and the 4 Hypothesized BI Predictors
aUTB refers to the Unified Theory of Behavior. bBehavioral Intentions (BIs) (range 0-10) refer to the strength of a providers self-described intentions to perform the specific behavior. cExpected-Values (range 0-10) refers to how likely a provider believes a positive or negative consequence will occur if they perform the specific ADHD assessment and/or treatment procedure. dDescriptive Norms refers to a PCPs best guess as to what percent of other PCPs are generally using the ADHD procedure. eOther Norms (range -5 to +5) refers to a PCP’s beliefs about whether his/her practice colleagues and important others would approve or disapprove of him/her applying the ADHD procedure. fSelf-Efficacy (range 0-10) refers to how easy a PCP feels it is to implement the specific ADHD practice.
Table 2: T1 and T2 UTB Factors Correlations with T2 Behavioral Intentions (T1 N=37; T2 N=38)
Note: T1 refers to the time frame immediately following training. T2 refers to six months post-training. UTB refers to the Unified Theory of Behavior. Expected-Values refers to how likely a provider believes a positive consequence will occur if they use ADHD assessment and treatment approaches. Descriptive Norms refers to a providers best guess as to how many other physicians are using the ADHD guidelines. Other Norms refers to how much a providers colleagues and people who are important to them would n=approve or disapprove of the provider applying the ADHD assessment and treatment approaches. Self-Efficacy refers to how easy a provider feels it is to implement the ADHD assessment and treatment approaches. *p<.10, **p<.05;
Over time, UTB predictors may change and lose power, such as when an PCP discovers a new behavior was harder than expected (thereby reducing self-efficacy), or encounters subsequent push-back on a new behavior from practice colleagues (practice norms), diminishing their initial predictive relationships with later BIs.
Table 3 analyses explored follow-up BI changes across time, CM status, and their interaction. Results revealed 4 significant effects of time, with 3 BIs increasing, and 1 decreasing. A review of the nature of those 3 increasing BIs suggests they began at lower levels, were somewhat more complex skills (weekly titration, switching to an alternate medication class, using higher doses), and might have been significantly benefitted by the ongoing training support provided during the 6 month’s bi-weekly conference calls. On the other hand, BIs for referring difficult patients to mental health services (#11) decreased significantly, perhaps because making such referrals was more difficult than expected. Of note, for this same behavior there was a significant effect of CM status, where PCPs with CM support showed more modest reductions over time, presumably because CM assistance enabled PCPs to be more successful with making mental health referrals.
Table 3: Effects of Time/Training and Case Manager Support on PCPs Behavioral Intentions
Other than this one exception, we found no significant effects of CM status, or CM x Time interactions. Reasons for this counter-intuitive finding are unclear, but the intensive nature of the initial training and the ongoing conference call support may have mitigated any further CM benefits due to ceiling effects. Alternatively, larger samples may be required to show possible CM effects. Multivariate regression analyses were also performed, entering CM status and T1-T2 changes in the 4 predictors into the model, examining their combined effects on T1-T2 changes in BI strength. As predicted by the UTB model, these analyses (available from the authors upon request) revealed that BI changes over time were significantly predicted by the combined T1-T2 UTB time changes in the 4 predictors (E-Vs, norms, self-efficacy) for 7 out of 11 Bis. CM status did not contribute significantly to any of the multivariate predictions. Of the 7 significant multivariate regressions, explained variance (R2) values ranged from 0.36 to 0.59 (adjusted 0.24 to 0.51). Of note, there was no evidence of multi-collinearity.
Because of the importance of the impact of perceived obstacles on PCPs performing new BIs, at study baseline we identified and measured ten common obstacles often cited by PCPs during similar, previous trainings (see left column of Table 4). We first computed zero order correlations with 10 of the 11 BIs for which the specific obstacles applied. Of 28 total zero order correlations (some obstacles applied to only several BIs), 18 were significant, indicating the importance of understanding, assessing, and planning to address relevant obstacles during the training process (analyses available from the authors upon request). Finally, to determine whether PCPs perceptions of obstacles changed as a function of the 6 months training or as a function of CM support, Table 4 presents analyses of the main effects of time (T1-2 changes), CM status, and their interaction. Significant improvements over time were found for 7 of the 10 obstacles, with all changes indicating substantial effects of time/training, resulting in meaningful decreases in PCPs perceived impact of obstacles. By way of contrast, main effects for CM status were found for only 2 obstacles, with both indicating reduced beliefs/concerns over time – #1) reduced concerns about time needed to conduct a thorough evaluation and #9) reduced concerns about family resistance to completing rating scales. No interactions between Time and CM status were noted.
Table 4: T1 to T2 Changes in Perceived Obstacles to Implementing Best Practices, By Time and Care Manager Status
Discussion
Overall findings indicate that UTB predictors of change (E-Vs, norms, and self- efficacy) are correlated with the central variable shown to predict behavior change (BIs), also found in hundreds of other basic science-guided studies. 25 We found the expected correlations between the UTBs predictor variables and BIs both cross- sectionally (Table 1) and prospectively (Table 2). The number of correlations seen in Table 1 indicate that E-Vs and self-efficacy are especially important for PCPs initial commitments/decisions to perform many but not all practice behaviors. For other, less common practices, such as #5 (obtaining parent/teacher rating scales during titration) and #6 (obtaining parent/teacher rating scales during follow-up visits), no E-V/BI correlations were found, but self-efficacy/BI correlations were. To offer an example of how such findings might be interpreted in the service of improving future trainings, from the perspective of a PCP such findings might mean, Im already certain of the value (E-V) of this well-recognized practice, but my commitment to do it (BI) is linked to how easy/hard I find it to be.
Should this interpretation be correct, an intervention designer might focus more during a future training to emphasize strategies that make such a practice easier, and possibly give participants more training and practice how to do it (self-efficacy). Table 2 deserves similar careful study. Inspection of the Table reveals that many correlations of Time 1 and 2 UTB variables (E-Vs, norms, self-efficacy) with T2 BIs increased from T1 to T2. Why might this happen? To illustrate, for #6, obtaining parent/teacher rating scales during ongoing monitoring, the correlation rose from 0.24 to 0.65. This finding could be explained because the initial BI was not strongly linked to any perceived value, but over time and after more experience, the PCP’s experience of its actual benefits on patient outcomes increased the BI. Of course, all these correlational findings deserve cautious interpretation, and newly developed hypotheses about the UTB underpinnings of BIs and actual behaviors will require newly designed, prospective, controlled studies.
Review of Table 3 indicates that time/training had important effects on multiple variables (#4, 7, 8, 10, 11). Of note, for #11, the main effect for time showed reduced BIs (making mental health referrals), but less so for the subgroup with CM support. This might suggest that the interest/willingness of PCPs (especially those without CM support) dropped substantially, as they attempted to perform the behavior but encountered difficulties. Within Table 3, we found no significant effects for 6 BI variables, but ceiling effects (BI scale range 0-10) were likely present for 4 (see BIs for #1, 2, 6, and 9), indicating PCPs’ nearly universal willingness/motivation/intentions to perform those behaviors at the completion of the initial training, and the strength of their intentions remained very high over 6 months. Future studies might be needed to determine whether sustained (6-months-long) training support for such high-BI behaviors is warranted, or if training resources should be devoted elsewhere. The lack of significant training impact on BIs 3 & 5 might indicate that future trainings should devote more resources to addressing/supporting those skills.
The multivariate regressions (tables available from the authors upon request) that examined changes in all 11 BIs as a function of the 4 predictor variables and CM status, highlight several important findings: First, the UTB model demonstrated robust predictions of 7 of 11 BIs over time, with sizable R2 values (ranging from 0.36 to 0.59). Second, CM support did not contribute significantly to any of the models. Third, the UTB model failed to be confirmed for 4 BIs (#5, 6, 9, & 10), but inspection of the individual UTB predictors for these BIs (Table 2) might suggest an operative mental phenomenon with PCP participants, no one else is doing it, so why should I?, and changes to future curricula for such BIs. Examining changes over time (Table 4) in PCPs perceptions of obstacles to new practices revealed that many initially anticipated obstacles dissipated over time – with training and ongoing support likely the essential ingredients in this process. All the time-related concerns (#1, 5, 6, 7) decreased significantly with experience, suggesting that this commonly perceived PCP concern is mitigated as PCPs are assisted to learn effective strategies for adopting those new behaviors. Two changes in obstacle perceptions were found for CM status: #1, time concerns about conducting an evaluation and #9, concerns about family resistance in using rating scales - both these procedures are areas where CMs often assist.
Further inspection of this table indicates that while only 7 of 10 obstacles showed significant reductions, trends in all 10 obstacles show numeric decreases over time, raising the possibility that a larger study sample would have generated additional significant findings. Although the current study offers support for the relevance of the UTB model for our understanding of the underlying mechanisms related to PCP practice changes, study limitations must be addressed. First, a relatively small number of providers participated in the study, likely rendering the study underpowered to detect some changes in variables where we found no effects. Thus, larger sample sizes will likely be needed to detect additional significant predictors of change, CM effects, and even further effects of time, including dissipation of training effects. Finally, although participants were randomly assigned to receive care management, additional research must examine the optimal level of CM intensity, and further explore how it interacts with PCP training support. Under what conditions are both necessary, and how does that vary with the training dose vs. the CM dose?
Conclusion
Several initiatives have been developed to aid in the likelihood that a PCP will adopt specific evidence-based strategies to improve the assessment, diagnosis, and treatment of ADHD among youth presenting to primary care settings. 10- 11, 39-45 The current study adds to this body of evidence by demonstrating the benefits of ongoing support to address PCPs personal barriers that might influence their adoption of new behaviors. The current study also lends support for the benefits of using an interactive and longitudinal training model that explicitly focuses on PCPs personal attitudes (E-Vs), norms, and self-efficacy beliefs that may influence their implementing practice guidelines.
Surprisingly, the current study did not offer much empirical support that including care mangers invariably helps promote the adoption of new ADHD practices, over and above the intensive six- month-long theory-guided intervention. However, this may be reflective of the research regarding collaborative care models more broadly. Specifically, systematic reviews on collaborative care and integrated behavioral health models reveals both successes for collaborative care approaches compared to other less intensive models and usual care, 46-47 but also with some mixed findings and failures to replicate. 48 Moreover, few rigorous studies (e.g., RCTs) have been conducted testing the benefits of various collaborative care models on childrens mental health outcomes. More specifically, to point us toward critical and urgently needed directions for future research, we have been unable to locate any studies examining and testing which ingredients in such models are effective. See the referenced reviews 45,48 for elucidations of different models and specific components that might be tested.
Pertaining to CMs, while they are often included in many (but not all) collaborative care models, it is possible that specific CM activities and intensities need further definition and adaptation to enhance treatment outcomes for pediatric populations. Because there appear to be no current studies (apart from this report) testing CM effects in the presence of a strong, theory-based intervention targeting PCPs behavioral practices, future studies should compare and contrast these two key elements to determine the extent to which either or both are necessary to produce changes in PCPs adoption of evidence-based ADHD practices.
Funding Source:
Funded by the National Institute of Health (NIMH), grant number RC1 MH088922. PIs: Peter S. Jensen, MD, and Lisa Hunter Romanelli, PhD Financial Disclosure: The authors have no financial relationships relevant to this article to disclose.
Conflict of Interest: Dr. Jensen receives book royalties from multiple publishing houses, is a shareholder of CATCH Services, LLC (a health consulting company), serves as the Board Chair of the REACH Institute (without compensation). The other authors have indicated they have no potential conflicts of interest to disclose.
Abbreviations: Attention-Deficit/Hyperactivity Disorder (ADHD), Primary Care Provider, (PCP), Unified Theory of Behavior (UTB), Behavioral Intention (BI)
Contributors Statement Page
Dr. Jensen conceptualized and designed the study, revised the initial draft, reviewed and revised the manuscript and all analyses, and approved the final manuscript as submitted.
Dr. Brandt drafted the initial manuscript, carried out the initial analyses, reviewed and revised the manuscript, and approved the final manuscript as submitted.
Dr. Kratochvil participated in study design, reviewed and revised the manuscript, and approved the final manuscript as submitted.
Dr. Humble participated in study design, provided day-to-day oversight of study personnel, reviewed and revised the manuscript, and approved the final manuscript as submitted.
Dr. Romanelli participated in study design, critically reviewed the manuscript, and approved the final manuscript as submitted.
Dr. Jaccard critically reviewed the manuscript and approved the final manuscript as submitted.
Acknowledgements:
We thank Marisa Domino, PhD, Lynn Wegner, MD, and Steven Wegner who contributed to the initial study design, and presented study findings at annual meetings of AcademyHealth and the American Public Health Association.
References
1. Stein, R. E., et al. Beyond ADHD: How well are we doing? Academic Pediatrics;2015.
2. Jensen PS, Hinshaw SP, Swanson JM, Greenhill LL, and the MTA Cooperative Group: Findings from the NIMH Multimodal Treatment Study of ADHD (MTA): Implications and Applications for Primary Care Providers. J Dev Behav Pediatrics 22:1-14, 2001.
3. American Academy of Pediatrics Committee on Quality Improvement Subcommittee on Attention-Deficit/Hyperactivity Disorder. Clinical practice guideline: Diagnosis and evaluation of the child with attention- deficit/hyperactivity disorder. Pediatrics. 2000;105:1158-1170.
4. American Academy of Pediatrics Subcommittee on Attention-Deficit/Hyperactivity Disorder Committee on Quality Improvement. Clinical practice guideline: treatment of the school- aged child with attention-deficit/hyperactivity disorder. Pediatrics;2001:108:1033-1044.
5. American Academy of Child and Adolescent Psychiatry. Practice parameters for the assessment and treatment of children, adolescents, and adults with attention- deficit/hyperactivity disorder. J Am Acad Child Adolesc Psychiatry;1997:36:85S-121S.
6. American Academy of Pediatrics Subcommittee on Attention-Deficit/hyperactivity Disorder Steering Committee on Quality Improvement and Management. ADHD: Clinical Practice Guideline for the Diagnosis, Evaluation, and Treatment of Attention-Deficit/Hyperactivity Disorder in Children and Adolescents. Pediatrics;2011:128.
7. Wolraich, M. L., Bard, D. E., Stein, M. T., Rushton, J. L., & OConnor, K. G. Pediatricians attitudes and practices on ADHD before and after the development of ADHD pediatric practice guidelines. J Atten Disord;2010:13:563-572.
8. Polaha, J., Cooper, S. L., Meadows T., & Kratochvil, C. J. The assessment of attention- deficit/hyperactivity disorder in rural primary care: The portability of the American Academy of pediatrics guidelines to the “real world”. Pediatrics;2005:115:e120-126.
9. Reiff, M., & Tippins, S. ADHD: A complete and authoritative guide. Elk Grove, IL: American Academy of Pediatrics; 2003. Brown, R.T., et al., Treatment of attention-deficit/hyperactivity disorder: overview of the evidence. Pediatrics;2005:115:e749-757.
10. American Academy of Pediatrics. Caring for Children With ADHD: A Resource Toolkit for Clinicians. 2nd ed. Elk Grove Village, IL: American Academy of Pediatrics; 2011.
11. Leslie, L. K., Weckerly, J., Plemmons, D., Landsverk, J., & Eastman, S. Implementing the American Academy of Pediatrics attention- deficit/hyperactivity disorder diagnostic guidelines in primary care settings. Pediatrics;2004:114:129-140.
12. Perkins, M.B., et al., Applying theory-driven approaches to understanding and modifying clinicians\' behavior: what do we know? Psychiatr Serv:2007:58:342-348.
13. Davis DA, Taylor-Vaisey A: Translating guidelines into practice; a systematic review of theoretical concepts, practical experience, and research evidence in the adoption of clinical practice guidelines. Can Med Assoc J 157:408- 416, 1997
14. Oxman AD, Thomson MA, Davis DA, Haynes RB: No magic bullets: a systematic review of 102 trials of interventions to improve professional practice. Can Med Assoc J 153:1423-1431, 1995
15. Bauer MS: A review of quantitative studies of adherence to mental health clinical practice guidelines. Harvard Review of Psychiatry 10(3):138-53, 2002
16. Cabana M, Rand C, Powe NR, et al: Why don’t physicians follow clinical practice guidelines? Journal of the American Medical Association 282(15):1458-65, 1999
17. Fox RD, Bennett NL: Continuing medical education: Learning and change: implications for continuing medical education. BMJ 316:466-468, 1998
18. Davis DA, Thomson MA, Oxman AD, Haynes RB: Changing physician performance: a systematic review of the effect of continuing medical education strategies. JAMA 274:700-705, 1995
19. Institute of Medicine, Committee of Quality of Health Care in America. Crossing the Quality Chasm. A New Health System for the 21st Century. Washington, DC: The National Academies Press; 2001. https://doi.org/10.17226/10027.
20. Katon W, Von Korff M, Lin E, Walker E, Simon GE, Bush T, Robinson P, Russo J: Collaborative management to achieve treatment guidelines: impact on depression in primary care. JAMA 273:1026-1031, 1995
21. Katon W, Robinson P, Von Korff M, Lin E, Bush T, Ludman E, Simon G, Walker E: A multifaceted intervention to improve treatment of depression in primary care. Arch Gen Psychiatry 53:924- 932, 1996
22. Soumerai SB, Avorn J: Principles of educational outreach (academic detailing) to improve clinical decision making. JAMA 263:549-556, 1990
23. Unutzer J, Katon WJ, Ming-Yu F, Schoenbaum MC, Lin EHB, Della Penna RD, Powers R. Long- term Cost Effects of Collaborative Care for Late- life Depression. Am J Manag Care 14(2): 95- 100, 2008.
24. Brettschneider C, Heddaeus D, Steinmann M, Haerter M, Watzke B, Koenig H. Cost- effectiveness of guideline-based stepped and collaborative care versus treatment as usual for patients with depression—A cluster- randomized trial. BMC Psychiatry Aug 28;20:427, 2020. doi: 10.1186/s12888- 020-02829-0
25. Davis R, Campbell R, Hildon Z, Hobbs L, Michie S. Theories of behaviour and behaviour change across the social and behavioural sciences: A scoping review. Health Psychol Rev 9:323-344, 2015.
doi: 10.1080/17437199.2014.941722
26. Bandura, A: Social Foundations of Thought and Action: A Social Cognitive Theory. Englewood Cliffs, NJ: Prentice Hall, 1986
27. Ajzen I, Fishbein M: Understanding Attitudes and Predicting Social Behavior. Englewood Cliffs, NJ: Prentice Hall, 1980
28. Ajzen I: The theory of planned behavior. Organ Behav Hum Decis Process 50:179-211, 1991
29. Janz NK, Becker MH: The health belief model: A decade later. Health Education Quarterly 11:1-47, 1984
30. Prochaska JO, DiClemente CC: Stages and processes of self-change of smoking: Toward an integrated model of change. J Consult Clin Psych 51:390-395, 1983
31. Rogers EM: Lessons for guidelines from the diffusion of innovation. Jt Comm J Qual Improv 21:324-328, 1995
32. Armitage CJ, Conner M: Efficacy of the Theory of Planned Behaviour: A meta-analytic review. British Journal of Social Psychology 40:471- 499, 2001
33. Fishbein, M: Developing effective behavior change intervention: Some lessons learned from behavioral research (pp. 246-261). In T.E. Backer, S.L. David, & G. Saucy (Eds.), Reviewing the behavioral science knowledge base on technology transfer. Rockville, MD: NIDA, 1995
34. Fishbein M, Triandis HC, Kanfer FH, Becker M, Middlestadt SE, Eichlar A: Factors influencing behavior and behavior change. In: Baum A, Revenson TA, Singer JE, eds. Handbook of Health Psychology. Mahwah NJ, Lawrence Erlbaum Associates; pp. 3-17, 2001.
35. Guilamo-Ramos, V., Jaccard, J., Pena, J., & Goldberg, V. (2005). Acculturation-related variables, sexual initiation, and subsequent sexual behavior among PuertoRican, Mexican, and Cuban youth. Health Psychology: Official J Div Health Psychol, American Psychological Association, 24(1), 88-95.
36. Guilamo-Ramos, V., Litardo, H. A., & Jaccard, J. Prevention programs for reducing adolescent problem behaviors: Implications of the co- occurrence of problem behaviors in adolescence. The Journal of Adolescent Health 36(1), 82-86, 2005
37. Jaccard J, Dodge, T., & Dittus, P. (2003). Maternal discussions about pregnancy and adolescents, attitudes toward pregnancy. The Journal of Adolescent Health. 33(2), 84-87.
38. Love AL, Jensen PS, Khan-Kapadia LA, Brandt TW, Jaccard J, for the PPP Steering Committee: The basic science of behavior change and its application to pediatric providers. Child and Adolescent Psychiatric Clinics. 26:851-874, 2017. doi: 10.1016/j.chc.2017.06.011.
39. Epstein, J. N., Langberg, J. M., Lichtenstein, P. K., Mainwaring, B. A., Luzader, C. P., & Stark, L. J. Community-wide intervention to improve the attention-deficit/hyperactivity disorder assessment and treatment practices of community physicians. Pediatrics;2008:122:19- 27.
40. Epstein, J. N., Langberg, J. M., Lichtenstein, P. K., Kolb, R., Altaye, M., & Simon, J. O. Use of an Internet portal to improve community-based pediatric ADHD care: a cluster randomized trial. Pediatrics;2011:128:e1201-1208.
41. Rushton, J.L., Fant, K.E. & Clark, S.J. Use of practice guidelines in the primary care of children with attention-deficit/hyperactivity disorder. Pediatrics;2004:114:e23-8.
42. Olson B.G., Rosenbaum P.F., Dosa N.P., & Roizen N.J. Improving guideline adherence for the diagnosis of ADHD in an ambulatory pediatric setting. Ambul Pediatr;2005:5:138- 142.
43. Epstein, J.N., et al. Improving Attention- Deficit/Hyperactivity Disorder treatment outcomes through use of a collaborative consultation treatment service by community- based pediatricians: A cluster randomized trial. Arch Pediatr Adolesc Med;2007:161:835- 840.
44. Epstein, J.N., Langberg, J.M., Lichtenstein, P.K., Kolb, R., & Simon, J.O. (2013). The myADHDportal.com Improvement Program: An inovative quality improvement intervetnion for improving the quality of ADHD care among community-based pediatricians. Clin Pract Pediatr Psychol;2013:1:55
45. Schlesinger A, Sengupta S, Marx L, Hilt R, Martini DR, DeMaso DR, Beheshti N, Borcherding B, Butler A, Fallucco E, Fletcher K, Homan E, Lai K, Pierce K, Sharma A, Earls M; AACAP Committee on Quality Issues (CQI), Rockhill C, Bukstein OG, Abright AR, Becker T, Diamond J, Hayek M, Keable H, Vasa RA, Walter HJ. Clinical Update: Collaborative Mental Health Care for Children and Adolescents in Pediatric Primary Care. J Am Acad Child Adolesc Psychiatry. 2022 Jun 6:S0890-8567(22)00291-X. doi: 10.1016/j.jaac.2022.06.001. Online ahead of print.
46. Xiao L, Qi H, Zheng W, Xiang Y-T, Carmody TJ, Mayes TL, Trivedi MH, Wang G. The effectiveness of enhanced evidence- based care for depressive disorders: a meta- analysis of randomized controlled trials. Transl Psychiatry. 2021; 11: 531. Published online 2021 Oct 16. doi: 10.1038/s41398-021-
01638-7
47. Gillies D, Buykx P, Parker AG, Hetrick SE. Consultation liaison in primary care for people with mental disorders. Cochrane Database of Systematic Reviews 2015, Issue 9. Art. No.: CD007193. DOI: 10.1002/14651858.CD007193.pub2.
48. Yonek J, Lee C-M, Harrison A, Mangurian C, Tolou-Shams M. Key components of effective pediatric integrated mental health models. JAMA Pediatr 174:487-498, 2020, doi: 10.1001/jamapediatrics.2020.0023.
Author Area
Have an article to submit?
Submission Guidelines
Submit a manuscript
Become a member