Home > Medical Research Archives > Issue 149 > Prediction of Drug Clearance in Preterm and Term Neonates by Minimal Physiologically Based Pharmacokinetic Model and Allometry: A Comparison with Whole BODY Physiologically Based Pharmacokinetic Model
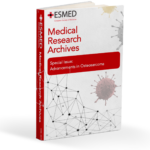
Published in the Medical Research Archives
Aug 2023 Issue
Prediction of Drug Clearance in Preterm and Term Neonates by Minimal Physiologically Based Pharmacokinetic Model and Allometry: A Comparison with Whole BODY Physiologically Based Pharmacokinetic Model
Published on Aug 30, 2023
DOI
Abstract
Objectives: The objective of this study is to compare the predictive performance of a minimal physiologically based Pharmacokinetic model (mPBPK) and an allometric model with a whole body physiologically based pharmacokinetic Model (PBPK) to predict clearance of drugs in preterm and term neonates.
Methods: From the literature, 6 studies were identified in which clearance of drugs in preterm and term neonates were predicted by whole body PBPK model. The mPBPK model consisted of four physiological parameters; liver and kidney weights and blood flow to these organs. From allometric models, the values of these physiological parameters were predicted in the neonates. The sum of these four physiological parameters were then used to predict CL in the neonates using adult CL values. The allometric model was based on Age Dependent Exponent Model (ADE). ADE uses different allometric exponents across the age groups. These exponents are 1.2 for preterm and 1.1 for term neonates for age 0-3 months, exponents 1.0, 0.90, and 0.75 for >3 months-2 years, >2-5 years, and >5 years, respectively. For the prediction of CL in the neonates from allometry adult CL values were used. The predicted clearance values using PBPK and mPBPK and ADE model were compared with the experimental (clinical) values. The acceptable prediction error was within 0.5-1.5-fold.
Results: There were 26 drugs with 86 observations. From PBPK, mPBPK, and allometric models, 90.7%, 98.8%, and 93.0% observations were within 2-fold prediction error, respectively. From PBPK, mPBPK, and allometric models, 81.4%, 90.7%, and 84.9% observations were within 0.5-2-fold prediction error, respectively.
Conclusions: This study indicates that the predictive performance of whole body PBPK, mPBPK and allometric models are essentially similar for the prediction of drug clearance in preterm and term neonates. mPBPK and allometry are much easier to develop than whole body PBPK and are as accurate and robust as whole body PBPK.
Author info
Introduction
Due to the physiological and biochemical differences between children and adults, it is important that a thorough consideration be given for the dosing of children (from preterm neonates to at least 12 years of age). Pediatric drug development requires a different strategy than adults since in children both safety and efficacy are the concerns because for ethical reasons children can only be dosed when they need medicine for an underlying disease1-3. On the other hand, first-in-adult dose is concerned with only safety and not necessarily efficacy but first- in-children dose requires both safety and efficacy1-3.
Drug development for pediatrics is important because pediatric diseases may differ from those of adults in terms of etiology, mechanisms, clinical or biological features, and the course of disease. The pharmacokinetics (PK) of drugs, in most instances, are different in children especially, in neonates than adults1-3.
It is possible to utilize modeling and simulation strategy to initiate a dedicated pediatric clinical trial. These models can extrapolate pediatric PK or dose from adults which can then be used with clinical experience and knowledge to initiate the pediatric clinical trial(s)1,4. Allometric, pharmacostatistical, and whole body or minimal physiologically-based models are widely used for the extrapolation of pediatric PK or dose from adults1, 3-9.
In order to predict pediatric PK or dose, whole body physiologically based-PK (PBPK) models have been suggested9-14. However, there are examples in the literature that suggest that the whole body PBPK model can be substantially simplified (reduced or minimal PBPK model) to predict PK parameters or dose of a drug15-19. Several studies have shown that allometric models predict pediatric PK or dose with fair degree of accuracy and are comparable with whole body PBPK20-28.
The prediction of PK or dose in preterm and term neonates is much more difficult than older children. The objective of this study is to predict clearance (CL) of drugs in preterm and term neonates by a minimal physiologically-based pharmacokinetic model (mPBPK) and allometry and compare the predictive performance of these two models with the whole body PBPK model.
Methods
DATA SOURCE FOR WHOLE BODY PHYSIOLOGICALLY-BASED-PHARMACOKINETIC MODEL (PBPK):
From the literature, 6 studies 6,10-14 were identified in which clearance of drugs for preterm and term neonates were predicted using PBPK model. From these studies, 26 drugs were found to be relevant to preterm and term neonates. Overall, clearance values for 26 drugs with 86 observations were obtained. In these studies, drugs were given to neonates either by intravenous or oral route. It should be noted that whole body PBPK models were not developed in this study. The reported predicted/observed ratios by the respective authors from the PBPK studies were used for comparison with the predicted/observed ratios obtained by mPBPK or allometry (this study). In a given study for PBPK, there were more drugs then analyzed in this study because with the exception of Abduljalil et al10 (only preterm neonates) the remaining studies consisted of older children and adolescents. Therefore, only those drugs were used from these studies which were for preterm and term neonates. Drugs were only repeated from one study to another if the age of the neonates (preterm or term) or/and route of administration was different from each other. This is explained in the footnote of Table 1.
METHOD I: MINIMAL PBPK MODEL:
For non-glucuronidated drugs the following minimal PBPK method was used. The first step in the development of this model was to predict the liver and kidneys weights as well as liver and kidneys blood flow in a given child as a function of body weight. Allometric model for these physiological parameters were developed using data from neonates to adults18. The following allometric equations were obtained for these parameters.
Liver weight in a child = 0.048*(body weight)0.847 (r2 = 0.990) (1)
Kidney weight in a child = 0.010*(body weight)0.807 (r2 = 0.987) (2)
Where; both body weight and organ weights are in kilograms.
Liver blood flow in a child = 0.088*(body weight)0.706 (r2= 0.990) (3)
Kidney blood flow in a child = 0.012*(body weight)1.121 (r2= 0.983) (4)
Where; blood flow is in liters/minute and body and organ weights are in kilograms.
From equations 1-4, organ blood flow rates and organ weights were determined in a child and each parameter was then divided by adult values. The adult values for liver weight, liver blood flow, kidney weight and kidney blood flow were 1.6 kg, 1.65 Liters/min, 0.3 kg, and 1.125 L/min, respectively18. The sum of all these four physiological parameters was then used to predict drug clearance in a neonate according to the following equation:
CL in a child = Adult CL * sum of the parameters* (weight of the child/70)0.75 (5)
METHOD II: MODEL BASED ON LIVER WEIGHT, LIVER BLOOD FLOW, AND URIDINE DIPHOSPHATE GLUCURONOSYLTRANSFERASE (UGT) ACTIVITIES:
There were some drugs in this study, which are metabolized by Phase II reaction mainly by glucuronidation. These drugs were midazolam, morphine, lorazepam, ibuprofene, and buprenorphine. For these drugs, the following minimal PBPK method was used as previously described by Mahmood22. This model consisted of several steps. Liver weight and liver blood flow in the neonates were predicted by equations 1 and 3. UGT activities in the neonates were predicted allometrically according to equation 622. The allometric model for UGT activities as fraction of adult activities were developed from pre-term neonates to 2 years old children22.
UGT activities in the neonate = 0.02*(body weight)1.45 (r2= 0.990) (6)
The sum of the liver weight, liver blood flow, and UGT activities were then used to predict drug clearance in a neonate according to equation 7:
CL in the neonate = Adult CL * sum of the parameters* (weight of the neonate/70) (7)
METHOD III. AGE DEPENDENT EXPONENT MODEL (ADE):
The ADE model employs variable exponents as a function of age8,20-28. In this method, different allometric exponents are used for different age groups and clearance is predicted in a given age group according to equation 8.
CL = Adult CL x (WC/W70)b (8)
Where the ‘adult clearance’ is the mean adult clearance of a given drug obtained from the literature. WC is the weight of a child and W70 is the weight of an adult standardized to 70 kg.
Exponent b in equation 1 is age dependent. The exponents used in equation 8 were 1.2 for preterm and 1.1 for term neonates for age 0-3 months, 1.0, 0.9, and 0.75, >3 months-2 years, >2-5 years, and >5 years, respectively. The exponents selected in the ADE model are based from previous experience, observation, and data analysis8, 20-27. Since in this study, only preterm and term neonates data were used therefore, the ADE exponents used in this study were 1.2 for preterm and 1.1 for term neonates. For drugs which are glucuronidated the exponents in equation 8 was 1.45 for the preterm neonates and 1.35 for term neonates22.
Statistical Analysis
The accuracy of the methods for the prediction of drug clearance was assessed by fold-error (FE) as the ratio of predicted to observed values:
FE = Predicted value/Observed value (9)
The following two categories of fold-errors were used: 0.5-1.5 (50% prediction error) and 0.5-2.0 (2-fold prediction error). In this study, a fold-error within 0.5-1.5 was considered reasonably accurate.
Results
There were 26 drugs and 86 observations (clearance values from different studies for the neonates). The adult CL values used in this study are provided in Table 1. In Table 2, predicted/observed ratios by PBPK, minimal PBPK, and allometry are presented. Overall results of the PBPK, mPBPK and allometric analysis of the studies are summarized in Table 3. A brief description of the results of each study is summarized below.
ABDULJALIL ET AL10
There were 6 drugs in this study in preterm neonates with 19 observations. The authors used a whole body PBPK model to predict drug clearances of these 6 drugs. From PBPK model, 100% and 78.9% observations were within 0.5- 2.0 and 0.5-1.5-fold prediction error, respectively. From minimal PBPK, 94.7% and 78.9% observations were within 2-fold and 0.5-1.5-fold prediction error, respectively. From allometric scaling, 84.2% and 78.9% observations were within 2-fold and 0.5-1.5-fold prediction error, respectively (Table 2).
Table 1: Adult clearance values used in the prediction of clearance in the neonates
Number in parenthesis is reference number.
*Midazolam oral CL based on 46% bioavailability (35)
For midazolam the neonates are preterm from reference 10 and term from reference 11. For gentamicin the neonates are preterm from reference 10 and term from reference 11. Midazolam was common for references 10 and 11 and it was considered as one drug.
Metronidazole was given as IV and oral therefore it was considered as one drug (reference 12). Theophylline was given as IV and oral therefore it was considered as one drug (references 11 and 12 ). Lorazepam is common in references 11 and 13 and were given IV to term neonates. Due to the substantial difference in the prediction of CL lorazepam by PBPK (reference 11 vs 13) was included in the analysis at two different places and was considered as one drug. In reference 11, the prediction error was >2-fold and in reference 13 the prediction error was <0.5-fold. `
EDGINTON AND WILLMANN11
There were 11 drugs in this study with 29 observations. The data were analyzed for both preterm and term neonates. Because the predicted/observed ratios for these drugs were not provided by the authors, these ratios were obtained from a previous study published by the same first author (9). From PBPK model, 89.7% and 86.2% observations were within 0.5-2.0 and 0.5-1.5-fold prediction error, respectively. From minimal PBPK, 100% and 93.1% observations were within 2-fold and 0.5-1.5-fold prediction error, respectively. From allometric scaling, 93.1% and 86.2% observations were within 2-fold and 0.5-1.5-fold prediction error, respectively.
UPRETI ET AL12
There were 8 drugs in this study with 12 observations. The data were analyzed for both preterm and term neonates. Out of 8 drugs, 4 were administered intravenously and 4 orally. From PBPK, mPBPK and allometry 100% observations were within 0.5-2.0-fold prediction error. From PBPK, minimal PBPK and allometry 83.3%, 91.7%, and 91.7% observations were within 0.5-1.5-fold prediction error, respectively.
VAN DER HEIJDEN ET AL13
Three drugs were analyzed (meropenem, ceftadizime, and lorazepam) in this study with 21 observations. The data were analyzed for both preterm and term neonates. From PBPK modeling, 76.2% observations were within 2-fold and 0.5- 1.5-fold prediction error. From mPBPK and allometry 100% observations were within 2-fold prediction error. From mPBPK and allometry 95.2 and 85.7% observations were within 0.5-1.5-fold prediction error, respectively. From PBPK, 4 observations were predicted with >2-fold prediction error (meropenem and ceftadizime), and one observation (lorazepam) was <0.5-fold prediction error. Prediction error from mPBPK and allometry remained within 0.5-2-fold prediction error for all observations.
Table 2: Predicted/observed ratio by PBPK, minimal PBPK, and allometry
DUAN ET AL14
There were 2 drugs (linezolid and emtricitabine) with 4 observations. The data were analyzed for both preterm and term neonates. From PBPK and mPBPK modeling, all observations were within 2- fold and 0.5-1.5-fold prediction error. From allometry, 75% observations were within 2-fold and 0.5-1.5-fold prediction error. The clearance of Linezolid was under-predicted with a prediction ratio of 0.45 by allometry.
ZHOU ET AL6
In this study, Sotolol was given to term neonates orally. The prediction error of CL in term neonates was within 0.5-2-fold by all three methods. The prediction error of sotolol by PBPK was 1.756 whereas, the prediction error by mPBPK and allometry was 0.60.
Overall, based on the analysis of 26 drugs and 86 observations, it was concluded that all three methods provided similar results (Table 3). For 26 drugs and 86 observations, 98.8%, 90.7%, and 93% drugs were predicted within 2-fold prediction error by mPBPK, PBPK, and allometry, respectively. There were 90.7%, 81.4%, and 84.9% drugs within 0.5-1.5-fold prediction error by mPBPK, PBPK, and allometry, respectively.
Table 3: Summary of the results by three methods (n = 86)
Discussion
Like adults, children also need medicine to manage or cure diseases but drug therapy in children is different than adults. The dosing recommendation of a drug in the pediatric population is generally based on the safety, efficacy, and pharmacokinetics data generated in adults. A normal practice is to scale the adult dose to infants and children based on body weight (per kg) or body surface area. This method however, does not take into account the effects of ontogeny on the efficacy and pharmacokinetics of drugs1-2.
Ontogeny is defined as “the history of the development of an individual from the fertilized egg to maturity”38. At least for the first decade of life, physiological changes occur rapidly but these changes are not a linear process. The adjustment of dosing in pediatric population based on body weight or body surface area without considering the developmental growth is inappropriate because body weight or body surface area does not really represent the true nature of overall organ function in the pediatric population39-41. The ontogenesis of clearance mechanism may be the most critical determinant of a pharmacological response in infants and children39-41. Numerous articles have been published outlining the developmental changes in children and need to predict drug clearance in children in order to select an optimal dose1,2.
Modeling and simulation is widely used in order to initiate a dedicated pediatric clinical trial. Although, there is uncertainty and the models are inherently erratic in nature, modeling is still a useful tool to predict pediatric PK parameters or dose right from the neonates to adolescents. The models can extrapolate pediatric PK or dose from adults which can then be used with clinical experience and knowledge to initiate the pediatric clinical trial(s)1,4. Such extrapolations can save time and cost of clinical trials which are initiated based on speculations or without any scientific basis. Allometric, pharmacostatistical, and whole body or minimal physiologically-based models are widely used for the extrapolation of pediatric PK or dose from adults1, 3-9,23. In this study, a comparative assessment of PBPK, mPBPK, and allometric modeling strategies was performed for the prediction of drug CL in preterm and term neonates. The overall results from 26 drugs and 86 observations indicate that the predictive performance of mPBPK and allometry is essentially comparable with that of whole body PBPK model for the prediction of drug clearance in preterm and term neonates.
In recent years, several studies compared whole body PBPK with allometry as well as with mPBPK15-19,8,21,22,28. The results of these studies indicate that both allometry and mPBPK provide comparative results with whole body PBPK. In recent days, mPBPK models have drawn a lot of attention mainly because it requires fewer physiological parameters and product characteristics 15-19. Mahmood developed several mPBPK models8,21,22,28 which are even simpler than the mPBPK models developed by other authors15-19. These simpler mPBPK models provide similar results as whole body PBPK models. The main characteristics of these simpler mPBPK models are that these models use only few physiological parameters which are associated with drug elimination such as liver and kidneys and blood flow to these organs. From these physiological parameters a factor was developed (equations 1- 7). Rather than generating concentration-time data to estimate CL in a target population, in mPBPK adult CL values are used which directly predict CL of drugs in the neonates. The entire process for the development of a mPBPK model is simple, does not require any sophisticated software, and is cost and time effective.
A whole body PBPK model uses a lot of physiological parameters and many product characteristics. Previously published papers by Mahmood8,21,22,28 and this paper indicate that one dose not really need every organ of the body and several characteristics of a product rather the entire method can be simplified and easily calculated on a spread sheet. PBPK model is one of the methods for modeling and like all other models carries errors and uncertainty. It is useful but it has its limitation and by no means PBPK or any other model should be used in lieu of a dedicated clinical trial.
A model may use physiological parameters but it does not mean that such a model represents a real physiological environment of a living organism. Few differential equations, several product characteristics in terms of molecular weight, pH, pKa, protein binding, blood to plasma ratio, and log P and even the inclusion of all the organs of the body in the model does not necessarily represent the true physiological system of a living organism. Simple mPBPK and empirical models such as allometry which do not represent the true physiological system of a living organism provide similar results as a whole body PBPK model because all these three models barely describe a true physiological system. A models predictive power may not be necessarily improved by adding a lot of parameters which may prove to be unnecessary. The comparative results between mPBPK and whole body PBPK indicates that many parameters used in whole body PBPK may be unnecessary. In this study, no product characteristics in terms of molecular weight, pH, pKa, protein binding, and blood to plasma ratio were used yet, the comparative results indicate that in a mPBPK model several product characteristics may not be needed. However, requirement of some product characteristics in terms of protein binding and/or log P values may be needed in mPBPK.
The comparable nature of the proposed method with whole body PBPK in this paper and previously published papers based on simplified version of mPBPK and allometry is strong evidence that despite public perception that a whole body PBPK will provide best results (since it is physiological) than other simple or empirical approaches, the whole body PBPK model can be substantially simplified and similar results can be obtained by these simple methods. There is also a perception that complex models provide better and accurate results than simple models. These perceptions are in fact, not correct.
The allometric principles can be applied to predict PK parameters such as clearance, volume of distribution, and half-life in pediatrics right from preterm neonates to adolescents8,20-28 and is of practical value during drug development. Allometric scaling based on body weight is easy and simple and can be done once PK information of a drug is available from adults. Allometric methods can also be applied for the projection of firs-in-pediatric dose23,24,26.
The conclusions of the studies and the method of allometric scaling vary depending on the age of the children. For example, Huisinga et al29 found that both clearance and volume of distribution at steady state (Vss) were predicted by allometry and PBPK with similar degree of accuracy. In this study, the age of the children was ≥5 years. In another study, Mahmood et al20 found that both allometry and PBPK provided similar results for the prediction of clearance in neonates and infants (≤3 months of age).
In a pharmacostatistical modeling, it is generally believed that allometry means a fixed exponent of 0.75 for clearance and 1.0 for volume of distribution. The application of exponent 0.75 has led to a substantial prediction error of clearance in pediatrics especially, in preterm and term neonates, infants, and toddlers30-33. This has further led to the wrong conclusions that allometry cannot be used to predict drug clearance in children ≤2 years of age. The allometric exponents are data dependent and widely vary and are based on several factors such as weight, age, and species30-
33. In neonates, infants, and toddlers, physiological changes develop very rapidly. Theses rapid physiological changes are nonlinear and a single exponent (linear allometry) cannot describe the clearance versus body weight or age across all age/weight groups1,8,32-34. In many instances non-linear allometry exists34. Due to this very fluctuating nature of the exponents of allometry, it is not surprising that one single exponent cannot predict drug clearance in pediatric population across all age groups. Therefore, different exponents are needed to describe such data.
In several previous studies, Mahmood suggested fixed but variable exponents (termed as age dependent exponents (ADE)) across the age groups20-28. Thus, by using a combination of allometric exponents, clearances of drugs can be predicted from preterm neonates to adolescents within 0.5-1.5-fold prediction error. In this study, ADE model was used for the prediction of CL in preterm and term neonates and 84.9% observations were within 0.5-1.5-fold prediction error and comparable with whole body PBPK (Table 3).
In this study and in some previous studies8,20-25,27,29, it was noted that the predictive performance of allometry was robust for the prediction of PK parameters and dose in children right from neonates to adolescents. When compared with PBPK, allometric model provided comparative results with whole body PBPK20,22-24. The reason for this is that most physiological parameters of living organisms are allometrically related with body weights. For example, the liver and the kidneys are the two major eliminating organs for xenobiotics. The allomretric exponents determined from neonates to adults for liver, kidneys, liver blood flow, and kidney blood flow were 0.847, 0.807, 0.706, 1.210, respectively (equations 1-4). These exponents are within the exponents of ADE model.
In a model known as body weight dependent exponent (BDE) model32,42-43 it was noted that the exponents of allometry for clearance vary across the age groups or body weight (neonates to adults). This change in allometric exponents as a function of age or body weight explains the allometric relationship with ontogeny or maturation for that particular age or body weight. The BDE model further supports the notion that a single exponent can not describe the CL vs body weight across the entire age groups and variable exponents are needed as shown in ADE model. ADE model can be considered a simpler version of BDE model. Further evidence that a single exponent can not describe the entire data from neonates to adults was supported by a segmented allometric model where entire data (neonates to adults) were described by different segments or exponents44.
Conclusions
This study indicates that mPBPK and allometry are robust and accurate enough (within 0.5-1.5-fold prediction error) to predict drug CL in preterm and term neonates. The predictive power of both these models is as good as a whole body PBPK model. Both these approaches are much simpler and easy to use than a whole body PBPK.
The proposed two methods can be used to estimate a first-in-pediatric (preterm and term neonates) dose during pediatric drug development based on the knowledge of observed adult clearance and predicted clearance in the pediatric population. The application of the proposed methods as well as whole body PBPK model is during drug development and is not in lieu of a pediatric clinical trial.
Conflicts of Interest Statement: There is no conflict of interest
Funding Statement: No funds were received from any source for this work
References
1. Mahmood I. Dosing in Children: A Critical Review of the Pharmacokinetic Allometric Scaling and Modelling Approaches in Paediatric Drug Development and Clinical Settings. Clin Pharmacokinet 2014;53:327-46.
2. Kearns G, Abdel-Rahman S, Alander S, etal. Developmental Pharmacology: Drug Disposition, Action, and Therapy in Infants and Children. N Engl J Med 2003;349:1157-67.
3. Abernethy D, Burckart G. Pediatric dose Selection. Clin Pharmacol Ther 2010;87:270-1.
4. Green D, Zineh I, Burckart G. Pediatric Drug Development: Outlook for Science-Based Innovation. Clin Pharmacol Ther 2018;103:376-8.
5. Rowland M, Peck C, Tucker G. Physiologically- based pharmacokinetics in drug development and regulatory science. Annu Rev Pharmacol Toxicol. 2011;51:45-73.
6. Zhou W, Johnson TN, Xu H, Cheung S, Bui KH, Li J, Al-Huniti N, Zhou D. Predictive Performance of Physiologically Based Pharmacokinetic and Population Pharmacokinetic Modeling of Renally Cleared Drugs in Children. CPT Pharmacometrics Syst Pharmacol 2016;5:475-83.
7. Edginton AN, Theil FP, Schmitt W, Willmann S. Whole body physiologically-based pharmacokinetic models: their use in clinical drug development. Expert Opin Drug Metab Toxicol. 2008;4:1143-1152.
8. Mahmood I. Prediction of drug clearance in premature and mature neonates, infants, and children ≤2 years of age: A comparison of the predictive performance of 4 allometric models. J Clin Pharmacol 2015; 56:733-39.
9. Edginton AN, Willmann S. Physiology-based versus allometric scaling of clearance in children: an eliminating process based comparison. Paediatr Perinat Drug Ther. 2006;7:146-53.
10. Abduljalil K, Pan Z, Pansari A, et al. Preterm Physiologically Based Pharmacokinetic Model. Part II: Applications of the Model to Predict Drug Pharmacokinetics in the Preterm Population. Clinical Pharmacokinet. 2020; 59:501-18.
11. Edginton AN, Schmitt W, Voith B, Willmann S. A mechanistic approach for the scaling of clearance in children. Clin Pharmacokinet. 2006;45:683-704.
12. Upreti VV, Wahlstrom JL. Meta-analysis of hepatic cytochrome P450 ontogeny to underwrite the prediction of pediatric pharmacokinetics using physiologically based pharmacokinetic modeling. J Clin Pharmacol 2016;56:266-83.
13. Heijden J, Feriksen J, Hoop-Sommen M, et al. Feasibility of a Pragmatic PBPK Modeling Approach: Towards Model-Informed Dosing in Pediatric Clinical Care. Clinical Pharmacokinet. 2022;61:1705-17.
14. Duan P, Fisher JW, Yoshida K,et al. Physiologically Based Pharmacokinetic Prediction of Linezolid and Emtricitabine in Neonates and Infants. Clin Pharmacokinet 2017;56:383-394.
15. Cao Y, Balthasar JP, Jusko WJ. Second- generation minimal physiologically-based pharmacokinetic model for monoclonal antibodies. J Pharmacokinet Pharmacodyn. 2013 (Epub ahead of print).
16. Cao Y, Jusko WJ. Applications of minimal physiologicallybased pharmacokinetic models. J Pharmacokinet Pharmacodyn. 2012;39:711- 23.
17. Björkman S. Reduction and lumping of physiologically based pharmacokinetic models: prediction of the disposition of fentanyl and pethidine in humans by successively simplified models. J Pharmacokinet Pharmacodyn. 2003;30:285-307.
18. Björkman S. Prediction of drug disposition in infants and children by means of physiologically based pharmacokinetic (PBPK) modelling: theophylline and midazolam as model drugs. Br J Clin Pharmacol 2004; 59:691-704.
19. Hardiansyah D, Ng CM. Effects of the FcRn developmental pharmacology on the pharmacokinetics of therapeutic monoclonal IgG antibody in pediatric subjects using minimal physiologically-based pharmacokinetic modelling. MAbs. 2018;10(7): 1144-1156.
20. Mahmood I, Tegenge MA. A Comparative Study Between Allometric Scaling and Physiologically Based Pharmacokinetic Modeling for the Prediction of Drug Clearance From Neonates to Adolescents. J Clin Pharmacol. 2019;59:189-197.
21. Mahmood I, Tegenge MA. Spreadsheet-Based Minimal Physiological Models for the Prediction of Clearance of Therapeutic Proteins in Pediatric Patients. Journal of Clinical Pharmacology 2021, 61(S1), S108- S116.
22. Mahmood I, Ahmad T, Mansoor N, Sharib SM. Prediction of clearance in neonates and infants (≤3 months of age) for drugs which are glucuronidated: a comparative study between allometric scaling and physiologically based pharmacokinetic modeling. J Clin Pharmacol. 2017;57:476-83.
23. Mahmood I. A comparison of different methods for the first-in-pediatric dose selection. Journal of Clinical and Translational Research 2022; 8:369-81.
24. Mansoor N, Ahmad T, Alam Khan R, Sharib SM, Mahmood I. Prediction of clearance and dose of midazolam in preterm and term neonates: a comparative study between allometric scaling and physiologically based pharmacokinetic modeling. Am J Ther. 2019;26: e32-7.
25. Mahmood I. Prediction of Clearance of Monoclonal and Polyclonal Antibodies and Non-Antibody Proteins in Children: Application of Allometric Scaling. Antibodies (Basel) 2020; 9, 40.
26. Tegenge MA, Mahmood I. Age- and Bodyweight-dependent Allometric Exponent Model for Scaling Clearance and Maintenance Dose of Theophylline From Neonates to Adults. Ther Drug Monit. 2018; 40:635-41.
27. Mahmood I. Prediction of clearance, volume of distribution, and half-life of drugs in extremely low to low-birth-weight neonates: An allometric approach. Eur J Drug Metab Pharmacokinet. 2017; 42:601-10.
28. Mahmood I. Prediction of total and renal clearance of renally secreted drugs in neonates and infants (≤3 months of age). Journal of Clinical and Translational Research 2022; 8:445-52.
29. Huisinga W, Solms A, Fronton L, Pilari S. Modeling interindividual variability in physiologically based pharmacokinetics and its link to mechanistic covariate modeling. CPT Pharmacometrics Syst Pharmacol. 2012 Sep 26;1:e4.
30. Calvier EA, Krekels EH, Välitalo PA, Rostami- Hodjegan A, Tibboel D, Danhof M, Knibbe CA. Allometric Scaling of Clearance in Paediatric Patients: When Does the Magic of 0.75 Fade? Clin Pharmacokinet. 2017;56:273-285.
31. Glazier D. Variable metabolic scaling breaks the law: from Newtonian to Darwinian approaches. In Proceedings of the Royal Society B: Biological Sciences. October 2022. 289: 20221605.
32. Wang C, Peeters MY, Allegaert K, van Oud- Alblas HJ, Krekels EH, et al. A bodyweight- dependent allometric exponent for scaling clearance across the human life-span. Pharm Res 2012;29:1570-81.
33. Mahmood I. Prediction of drug clearance in preterm and term neonates: different exponents for different age groups? In: Pharmacokinetic allometric scaling in pediatric drug development. Rockville: Pine House Publishers; 2013; p. 88-100.
34. Knell JM. On the analysis of non-linear allometries. Ecological Entomology 2009; 34, 1-11.
35. Heizman P, Eckert M, Ziegler W. Pharmacokinetics and bioavailability of midazolam in man. Br. J Clin Pharmacol 1983;16:43S-9S.
36. Martin W, Koselowske G, Töberich H, Kerkmann T, Mangold B, Augustin J. Pharmacokinetics and absolute bioavailability of ibuprofen after oral administration of ibuprofen lysine in man. Biopharm Drug Dispos 1990;11:265-78.
37. Golper T, Noonan H, Elzinga L et al. Vancomycin pharmacokinetics, renal handling, and nonrenal clearances in normal human subjects. Clin Pharmacol Therap. 1988;43:565-70.
38. The Bantam Medical Dictionary (2nd edition). New York, Bantam Books, 1996;313.
39. Alcorn J, McNamara PJ. Ontogeny of hepatic and renal systemic clearance pathways in infants: part I. Clin Pharmacokinet 2002;41:959-98.
40. Alcorn J, McNamara PJ. Ontogeny of hepatic and renal systemic clearance pathways in infants: part II. Clin Pharmacokinet 2002; 41:1077-94.
41. Kanamori M, Takahashi H, Echizen H. Developmental changes in the liver weight- and body weight-normalized clearance of theophylline, phenytoin and cyclosporine in children. Int J Clin Pharmacol Ther 2002;40:485-92.
42. Wang C, Allegaert K, Peeters MY, Tibboel D, Danhof M, Knibbe CA. The allometric exponent for scaling clearance varies with age: a study on seven propofol datasets ranging from preterm neonates to adults. Br J Clin Pharmacol 2014;77:149-159.
43. Bartelink IH, Boelens JJ, Bredius RG, Egberts AC, Wang C, et al. Body weight-dependent pharmacokinetics of busulfan in paediatric haematopoietic stem cell transplantation patients: towards individualized dosing. Clin Pharmacokinet 2012; 51:331-45.
44. Mahmood I, Staschen CM, Goteti K. Prediction of drug clearance in children: an evaluation of the predictive performance of several models. AAPS J 2014; 16:1334-43.
Author Area
Have an article to submit?
Submission Guidelines
Submit a manuscript
Become a member