Home > Medical Research Archives > Issue 149 > Mask-Wearing Onboard Airplanes During COVID-19 - Identifying Passenger Segments Using Cluster Analysis
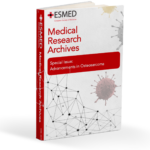
Published in the Medical Research Archives
Aug 2023 Issue
Mask-Wearing Onboard Airplanes During COVID-19 - Identifying Passenger Segments Using Cluster Analysis
Published on Aug 31, 2023
DOI
Abstract
The COVID-19 crisis has had an unprecedented impact on air traffic, but its long-term effect on how people view and conduct air travel could be more significant. While some studies have identified factors that can affect the perception of COVID-19 and behaviors in transportation, few have examined factor impact at the segment level, especially with respect to mask-wearing as a protective measure onboard airplanes during COVID-19. This study fills the research gap by identifying passenger segments with different perceptions of mask use onboard airplanes, and which factor in the theory of planned behavior (TPB) model can be best distinguished in the different segments. Survey data was collected from MTurk for cluster analysis, which considered the comfort of mask-wearing, risk avoidance, and information-seeking jointly as the cluster variates. The analysis led to the formation of three passenger segments: 1) Comfort First – most attention was paid to the comfort of mask-wearing; 2) Risk Avoider – the greatest importance was given to avoiding the risk and searching for information about COVID-19; and 3) Balanced Group – having a balanced view on the importance of the three cluster variables. The multinomial logistic regression analysis showed that the three passenger segments differed most in their attitude toward masks, suggesting that attitude, compared to the other two TPB constructs (subjective norms and perceived behavioral control) can best predict the cluster membership of air travelers with regards to mask-wearing onboard airplanes. The findings provide useful guidance for the airline industry to recover safely and effectively from the COVID-19 pandemic, and may aid in preparations for future pandemics.
Author info
Introduction
The rapid spread of COVID-19 has dramatically reduced air travel demand both domestically and internationally. The impact is particularly strong in the US where COVID-19 led to more damages so far, compared to other countries, which significantly reduced domestic passenger traffic.1 To encourage air travel during COVID-19, airlines have taken protective measures to ensure the health and safety of air travelers, focusing on mask-wearing. The success of any protective measure to slow the spread of COVID-19 relies extensively on the attitudinal and behavioral change of individuals.2 Unlike other measures like social distancing and handwashing, mask-wearing has received mixed public acceptance despite numerous studies supporting their effectiveness in reducing COVID- 19 transmission.3,4,5 In fact, mask-wearing has been a highly controversial issue leading to varied levels of compliance, especially in countries such as Canada and the US where face covering is not a tradition.
Significant literature has investigated the influence of factors on the intention to comply and behavior regarding mask-wearing in COVID-19, indicating that risk perception of the disease and attitude toward mask-wearing, among other factors, were significant.6,7 While these studies have enhanced our understanding of mask-wearing behaviors during COVID-19, they were mainly focused on individual factors and failed to consider that intentions and behaviors in the context of a highly transmissible disease are often influenced by multiple factors working together. Mask-wearing during COVID-19 is the result of a complex decision influenced by the combined effect of psychological, sociological, and economic factors, and their interactions, which have not been fully explored in the context of transportation, especially in the aircraft cabin where the risk of infection can be high. It also remains unknown whether certain passenger segments demonstrate specific mask-wearing characteristics. Due to the complexity of the decision to wear a mask, air travelers may have disparate views about mask use in airplanes and by identifying such views, airlines can better understand and protect their customers during global pandemics. This study examined passenger composition and mask-wearing onboard airplanes, while considering multiple factor influences. It further identified the factors that can best discriminate between the different passenger groups.
Literature Review
APPLICATION OF CLUSTER ANALYSIS IN COVID-19 STUDIES
Cluster analysis is a multivariate statistical technique for grouping objects into distinct categories based on their characteristics.8 These characteristics of the objects, referred to as clustering variables, are often determined by the researcher following comprehensive literature review. If the classification is successfully performed, the resulting clusters should demonstrate high homogeneity within clusters and high heterogeneity between clusters.8 By revealing the patterns among objects in a dataset, cluster analysis allows the researcher to identify the underlying structures that may not be apparent before, providing meaningful evidence for informed decision-making. Cluster analysis has been used in many research areas, including transportation.9,10,11 Recent studies have used cluster analysis to define the structure of data related to COVID-19, in areas such as hospital capacity investigation,12 utility consumption,13,14 buying behaviors,15,16 information and social media influence,17,18,19 and public perception and compliance behaviors,2 to name just a few.
One of the research interests is to understand similarities and differences in COVID-19 coping behaviors. Kleitman et al.,2 for example, collected respondent views from Australia, Canada, the US, and the UK to classify respondents into the compliance and non-compliance groups regarding protective behaviors in COVID-19.2 They further revealed group differences in attitude, personality, political, cultural factors, among other factors. Another study applied cluster analysis to identify consumer segments based on COVID-19 pandemic perception and responses in the US.20 Two data collections, conducted nine months apart, led to different segmentations of consumers. Specifically, the study identified a three-cluster solution (Prepared, Apprehensive, and Dismissive) early in COVID-19 and a two-cluster solution (Dedicated and Dismissive) later in the pandemic, suggesting different patterns in COVID-19 response at different stages of the pandemic. Typological studies examining consumer behaviors in COVID-19 have also been conducted in Southeast Asia. Hartono et al.15 identified adaptive shopping patterns in Indonesia during COVID-19. Their study formed five clusters - rational buyers who reduced and shifted consumption, non-panic buyers who adapted well to the pandemic environment, wealthy young buyers who were not price sensitive, minimum adapters who were poorly adjusted to the new consumption environment, and price sensitive buyers who were low-income buyers - demonstrating heterogeneity in consumption behaviors during a global pandemic.
Surprisingly, only limited studies applied cluster analysis in the research of transportation during COVID-19 despite the fact that transportation, especially air transportation, may contribute to fast spread of COVID-19 and travelers often exhibit different characteristics and coping behaviors during the pandemic. Indeed, coping intentions and behaviors of air travelers in COVID-19, especially at the segment level, have been substantially understudied. Two studies in transportation used cluster solutions to understand travelers. Kopsidas et al.21 investigated anticipated, post-pandemic behaviors of travelers toward public transport use in Greece. The cluster analysis revealed four distinct groups – students, low-income frequent travelers, high-income non-frequent travelers, and low-income, non-frequency travelers. It further indicated that socio-demographic characteristics and psychological factors significantly influenced public transport recovery time following the pandemic. Only one study applied cluster analysis to air transportation, focusing on identifying segments among passengers in Norway based on their shared attitudes and preferences of air travel during COVID-19, as well as their intention to fly in the future.22 Three cluster variables (air travel in COVID-19, concerns of airport experience, and concern of onboard airplanes) were used to generate four passenger clusters including Cautious Commuters, Apprehensive Elder, Intrepid Explorers and Sanguine Shielders, each exhibiting different attitudes, behaviors, and levels of concern about air travel.
It was found that all segments preferred active protective measures such as social distancing and mask-wearing over other interventional measures. These studies, while shedding useful insight on shared characteristics of COVID-19 response in transportation, were not directly related to airline passengers and their mask-wearing intentions and behaviors during a global pandemic. The literature review indicated a significant gap in the research of passenger characteristics and coping behaviors in global pandemics, especially regarding mask-use on airplanes. Face masks, unlike social distancing and handwashing that received universal acceptance, have generated mixed feelings and attitudes. This is especially true in the US where public views of mask-wearing vary extensively. For academia and industry alike, a better understanding of air travelers’ mask perceptions and the varied groups they make up is needed, especially given the scarce literature in this area of research. While many factors such as risk avoidance and attitude have been found to be important for mask use, they are usually assessed as individual contributors, which fails to recognize the complexity of the decision to wear a mask and how the factors might influence each other. Cluster analysis can add value to this understanding given its ability to reveal group patterns among airline passengers based on their shared characteristics regarding mask use onboard airplanes. Because the technique has not been used fully in this context, this study aims to bridge the research gap by applying a two-stage cluster analysis, with risk avoidance, comfort, and information-seeking as the cluster variables, to uncover the different groups with respect to mask-wearing onboard airplanes during COVID-19.
SELECTION OF CLUSTERING VARIABLES
The success of cluster analysis relied heavily on the selection of cluster variables that can best categorize objects in terms of their similarities and differences. As cluster analysis provides no method for detecting the relevancy of cluster variables, the researchers judgement is essential in choosing variables that have the strongest theoretical support to provide multidimensional characterization of the objects been clustered.8 This study selected three variables – comfort, risk avoidance, and information-seeking with respect to mask use onboard airplanes to identify airline passenger segments in COVID-19. They are suitable cluster variables because they are measured numerically and have the same common foundation closely related to mask-wearing in COVID-19, as frequently suggested in the literature. In this study, comfort of mask-wearing referred to physical and psychological ease and relaxation of air travelers when they wear masks onboard airplanes during COVID-19. Face masks are perceived by many as uncomfortable, which may contribute to mask reluctance. The discomfort of masks and its possible impact were reported in Gray et al.23
It was found that comfort significantly factored in people’s view of mask use in New Zealand, with 40% of the survey participants strongly feeling the discomfort of mask-wearing. The study concluded that barriers to mask use were less related to cost and embarrassment but more to other factors such as comfort.23 While comfort was among the most common perceptions of mask use, it may not have a direct relationship with actual mask use.24 In other words, comfort in mask-wearing by itself may not always trigger the actual behavior of mask-wearing, suggesting that comfort should be considered in conjunction with other factors in assessing mask behaviors during COVID-19. This was supported by Cheok et al.,25 which found that mass mask compliance can be achieved despite the significant discomfort perceived by people in Singapore, citing positive public attitude as a significant contributor.
The relationship between risk factors and health- related behaviors has been widely studied, generally suggesting a strong relationship.26 This relationship was found to be significant in the US during COVID-19, even though Americans have different opinions of the risks associated with COVID-19 at different stages of the pandemic.27 Those who perceived higher risks of COVID-19 were more likely to engage in protective behaviors, especially in the later stage of the pandemic compared to the early one. A positive relationship has also been found between mask-wearing and perceived risks of COVID-19. Irfan indicated that willingness to wear a mask in Pakistan was significantly affected by attitudes, social norms, risk perceptions of the pandemic, and perceived benefits of face masks, with risk perception generating the strongest influence.6 Thus, the risk factor of COVID-19 is highly relevant to this study, given the transmissibility of the virus onboard airplane and the effect of masks to mitigate the risks, both can influence mask behaviors.
The risk perception of COVID-19 is shaped largely by publicly available information, which can also influence the willingness to engage in protective behaviors during the pandemic. Given the mixed attitudes toward face masks especially in the US, information searching of masks may change COVID-19 risk perception, which can complicate public health messaging about mask-wearing.28 The inter-relationship of information-seeking, COVID- 19 perception, and coping strategy has been identified, with studies generally supporting a significant relationship among these factors.29,30 especially regarding the negative impact of misinformation on COVID-19 responses and behaviors.31 Recent studies have also applied cluster analysis to identify patterns of information presented in different sources and how people may react differently to these information patterns.17,18 In Lang et al.,19 the cluster analysis identified four clusters based on information of news and social media in addition to trust issues, with each cluster demonstrating different demographic, attitudinal, and behavioral characteristics.19 Despite the importance of information searches in shaping COVID-19 perception and behaviors, the information factor has not been used as a cluster variable in the COVID-19 studies, especially in the area of transportation.
FACTORS THAT DISCRIMINATE BETWEEN CLUSTERS
Following the identification of clusters, it is important that researchers profile the cluster solution through describing the characteristics of each cluster on variables that are not included in the cluster variables.8 In the current study, three constructs of the theory of planned behavior (TPB) model – attitude toward masks, subjective norms, and perceived behavioral control (PBC) were used to find out if they differed across the clusters and, more importantly, which one of them was the key distinguishing factor that can be used to discriminate between the clusters. The selection of the variables for this purpose requires strong theoretical support, which can be found for the three TPB factors. The original constructs of the TPB model, attitude (the psychological favorable or unfavorable feeling toward a behavior), subjective norms (the expectations from important others for one to conduct a behavior), and the PBC (the perceived ease or difficulty in engaging in a behavior) have been used to predict intentions and behaviors in a wide range of contexts.32,33,34 including mask- wearing during COVID-19.6,35
Attitude is a relatively stable psychological predictor of mask-wearing intentions and behaviors during COVID-19. Studies routinely found that positive attitude contributed to greater mask compliance despite the discomfort associated with mask-wearing,25 while a range of anti-mask attitudes were associated with mask refusal.36 As such, attitude is often considered a prerequisite for mask intentions and behaviors. This was evident in the positive attitudinal impact on mask-wearing intentions of urban and rural populations in China,37 the mask-saving behaviors in the post-pandemic era,35 and international students’ intention to wear masks during COVID-19.7 Social norms can provide common standards in a society regarding socially desirable behaviors, including health behaviors such as mask-wearing in COVID-19.38 Social norms can often be a powerful force of influence, as they can trend public behavior in the appropriate direction, even in the absence of government intervention. Such influence was found in Barcelό and Sheen which indicated a positive relationship between social norms and mask uptake especially among elderly population, even in a country with no mask culture.39 The perceived ease or difficulty in completing a task is another important driver of mask intention and behavior. Studies found that perceived behavioral control, along with attitude and subjective norms, significantly influenced mask use among leisure activity participants and post- pandemic mask-saving intentions.31,35
It should be noted that, while attitude, subjective norms, and perceived behavioral control were often important factors in mask behaviors, the factor impact varied in the COVID-19 studies6, 7,31,37,40 Given the mixed findings of attitude, subjective norms, and perceived behavioral control on mask use, it is necessary to examine further the impact of the three TPB constructs. The investigation is especially important at the segment level, where the predictive ability of these factors on group patterns of air travelers\\\' mask-wearing has not been tested. Finally, while attitude was often an important factor in mask-wearing, some studies suggested different results.6 Given the mixed findings of attitude, subjective norms, and perceived behavioral control on mask use, it is necessary to examine further the impact of the three TPB constructs. The investigation is especially important at the segment level, where the predictive ability of these factors on group patterns of air travelers’ mask-wearing has not been tested.
Methodology
SAMPLING AND DATA COLLECTION
This study collected survey data of American air travelers using Amazon Mechanical Turk (MTurk). MTurk for data collection is used because (1) given the pandemic situation, online survey provided a feasible way to obtain data for this study, and (2) MTurk is able to provide a sample that is often more representative of the US population compared to other convenience sampling approaches.
Data collection was conducted in May 2021 when vaccination started to show a positive effect in the US. Despite the success of the vaccine, however, COVID-19 continues to bring uncertainty to public health especially with its new variants that can be potentially more transmissible. Many people, even fully vaccinated, may still prefer to err on the side of caution, continuing to wear masks in crowded settings especially in the aircraft cabin where social distancing is not possible. When making inflight mask decisions during this transitional period (vaccination would accelerate the removal of mask mandate, yet uncertainty of COVID-19 remains), air travelers are likely to simultaneously evaluate multiple factors such as risks of infection, comfort of mask-wearing, and information they obtained and believed to be true. Conducting the survey at this time allowed the researcher to better understand air travelers’ views of the importance of these factors in their mask use decisions.
SURVEY INSTRUMENT
A survey questionnaire was developed to collect data on MTurk. The first two sections of the questionnaire collected information of passenger demographics and travel experience. Section Three was scenario-based for respondents to evaluate the factors that could drive mask use on airplanes during COVID-19. As this study aimed to investigate voluntary use of face masks during flight, respondents were given a scenario in which they would imagine taking a flight in the next three months when airlines started to remove mask mandate on airplanes, yet COVID-19 continued to bring uncertainty to air travelers, both physically and psychologically. Respondents were then asked to evaluate how factors including comfort of masks, risk avoidance, information-seeking, attitude toward mask, subjective norms, and perceived behavioral control contributed to their decision to voluntarily wear masks during flight on a five-point Likert scale from 1 (strongly disagree) to 5 (strongly agree). Scale items measuring these factors were mostly adopted from the literature to ensure the validity of measurement.30,33,41,42,43,44,45,46,47,48,49,50, 51 Table 2 and Table 3 in Section 4.1 shows the
profile of the respondents.
DATA ANALYSIS
Three sets of data analysis were conducted on the entire sample (n=1,121) to answer the research questions. First, a two-stage cluster analysis was performed to group similar observations based on the characteristics they possessed, using both hierarchical clustering and non-hierarchical clustering algorithms. A major challenge associated with clustering is that the number of clusters within the data is often unknown beforehand. To address this issue, hierarchical clustering was used in Stage One to exam a wide range of cluster solutions and identify the most suitable number of clusters, based jointly on the three cluster variables – comfort of mask, risk avoidance, and information-seeking. The number of clusters (groups) identified in this process was then analyzed by non-hierarchical clustering procedure (Stage Two) to determine the final cluster characteristics.8 The second analysis profiled the clusters identified in the first analysis, using variables not included in the cluster variates. The profiling used thirteen demographic, travel, and mask-related variables collected in the survey to find out if the identified clusters exhibited differences on these variables, in order to validate the clusters. The third analysis was predictive in nature, using attitude, subjective norms, and perceived behavioral control to predict observations being grouped into specific clusters. Different from the second analysis, the third analysis attempted to understand the variables that could impact on the formation of clusters. For this purpose, multinominal logistic regression was performed to identify if attitude, subjective norms, and perceived behavioral control differed across the clusters, and which one of them demonstrated the strongest predictive power on group patterns of mask- wearing in the context of flying during COVID-19.
Results
CLUSTER ANALYSIS
A two-stage cluster analysis was conducted using both hierarchical and non-hierarchical techniques. First, hierarchical clustering was used to identify a small subset of possible cluster solutions given the data, from where the researcher selected the most appropriate number of clusters to be used for grouping respondents based jointly on the three cluster variates - comfort, risk avoidance, and information-seeking regarding mask use onboard airplanes during COVID-19. The optimal number of the clusters was determined based on two diagnostic measures – change in agglomeration coefficient and evaluation of dendrogram. An agglomeration coefficient measures the structure in the dataset based on similarity and dissimilarity of the observations being clustered. As small coefficients indicate the merging of homogeneous clusters and large coefficients indicate joining two very different clusters, the researcher should focus on large percentage changes in the coefficient to determine the stopping rules.8 As shown in Appendix A, the largest percentage increase, 81.85%, was associated with moving from Stage 1119 to 1120 (two to one cluster), followed by 41.67% which represented moving from Stage 1118 to 1119 (three to two clusters), suggesting the possible use of two or three clusters in this study. The dendrogram, a tree-like structure that visually displays the relations between all data points, produced similar results of using two or three clusters. Although 81.85% was the largest increase in coefficient, the selection of the final cluster solution must also consider the objective of the study.
Given the largely different views in the US regarding risk perception, comfort, and information of mask-wearing in COVID-19, a two-cluster solution may lack the capacity of capturing meaningful combinations of factor impact, thus may only represent limited value in meeting the research objective. This can be clearly seen in the graphical comparison of the two- and three-cluster solutions in Appendix A. Cluster 1 in the two-cluster solution is almost identical to Cluster 3 in the three-cluster solution, suggesting that this group of respondents had characteristics that can be easily identified by both cluster solutions. Cluster 2 in the two-cluster solution, however, shows similar weights of all cluster variables, indicating similar impact of these variables on respondents in this group. With a three-cluster solution, however, this group was further segmented to reveal distinct patterns among these respondents (See Clusters 1 and 2 of the three-cluster solution). Because the three-cluster solution can better identify patterns in the data, it is considered the optimal cluster solution to meet the goal of this study. The researcher then performed non-hierarchical clustering (Stage Two of cluster analysis), using the K-means optimizing algorithm to re-assign observations to the three clusters until maximum homogeneity within clusters was achieved.8
Table 1. Three Cluster Solution Based on Comfort, Risk Avoidance, and Information-Seeking
Table 1 shows different mean scores of the cluster variables across the three clusters, indicating that the cluster solution can adequately discriminate between observations. The uniqueness of the cluster variables was supported by the variance inflation factor (VIF) scores associated with each cluster variable (< 2), which indicated minimal concern of multicollinearity. The patterns observed in the cluster means were used to label the clusters. Cluster One was the smallest group, containing 150 respondents (13% of the total sample).
Compared to the other clusters, Cluster One travelers assigned the highest score to comfort of mask-wearing during flight, and the lowest scores to risk avoidance and information-seeking. Hence, the name “Comfort First” was used to label this cluster. Cluster Three was almost the exact opposite of Cluster One. The largest cluster consisting of 550 respondents (49% of the total sample), this group was most distinguished by the highest scores assigned to risk avoidance and information- seeking, and the lowest score to comfort of mask-wearing. Hence, the name “Risk Avoider” best described this cluster. Cluster Two was positioned in the middle of the two extreme clusters. The second largest group with 421 respondents or 38% of the total sample, Cluster Two paid most attention to risk avoidance, but the scores assigned to the three variables in this group were rather average compared to the other two clusters. Thus, this group can be described as “Balanced Group” with respect to their views on mask use. The three clusters were statistically different, indicating the usefulness of segmenting air travelers into three clusters regarding their views on mask use on airplanes during COVID-19.
VALIDATING AND PROFILING THE CLUSTERS
Given the exploratory nature of cluster analysis, it is important to validate the cluster solution by profiling the clusters on additional variables not used throughout the clustering process. Thirteen ordinal-categorical variables were used to test the difference across the three clusters. The Chi-square analysis was performed on the relationship between these variables and the cluster membership. Significant Chi-Square values were observed for ten of the thirteen profile variables, generally supporting the difference between the clusters.
The Comfort First cluster contained an equal number of male and female travelers, with majority of them (61%) aged between 30 and 50 years old. This group had the highest number of low-income travelers (15% below $25,000 annually) among the three clusters, while 53% earned an annual income between $25,000 and $75,000. Comfort First travelers were comprised predominantly by White travelers (87%), the highest percentage of the three clusters. The most reported travel frequency before COVID-19 was 2-3 times (37%), while during COVID-19 less than one time became the most reported (38%). Travelers in this cluster almost never wore masks before COVID-19 in crowded settings such as supermarkets and conferences (1%), and their mask use remained the lowest of the three clusters in these settings during COVID-19 (79%). Their mask behaviors appeared to be related to their negative views of masks. Only 12% and 22%, respectively, in this group believed masks can protect the wearer and others. Not surprisingly, only 8% of Comfort First travelers chose to voluntarily wear masks onboard airplanes during COVID-19, far lower than the other two clusters.
Cluster 3, Risk Avoider, showed a vastly different profile. This group contained more females than males (57% vs. 43%), mostly young and mid-aged. Travelers in this group was generally more educated compared to Comfort First travelers, and the most reported annual incomes were between $25,000 to $75,000. White travelers accounted for the majority in this group (75%), although the percentage was much lower compared to Comfort First group. Forty-four percent of Risk Avoider travelers traveled 2-3 times before COVID-19, the largest category. reported of all clusters. During COVID-19, however, over half of the group chose not to travel, again the largest category across all clusters. While very few Risk Avoider travelers (4%) wore masks in crowded settings before COVID-19, almost all of them wore masks during COVID-19 (97%). This group strongly supported the effect of masks, believing mask- wearing protects the wearer (93%) as well as others (99%). When giving an option, 95% of Risk Avoider travelers would choose to wear masks onboard airplanes during COVID-19, far more than the other two clusters.
Balanced Group (Cluster Two), as the name implied, exhibited moderate differences regarding the profiling variables. With an equal gender mix, this group consisted mostly of younger travelers between 21 to 40 years old (61%) and showed higher education compared to the other two clusters. Most reported annual incomes, again, fell between $25,000 and $75,000. While White travelers were still the majority (70%), the percentage is the lowest of the three clusters. Regarding travel frequency, 2-3 times were most reported for travel before COVID-19 (38%), and less than one time was most selected since the start of the pandemic (36%). A considerable amount of Balanced Group travelers wore masks in crowed settings even before COVID-19 (28%), which increased to 95% during COVID-19. Majority of the group believed that masks protected themselves and others (84% and 92%, respectively). If given an option, 83% of the group would choose to wear masks onboard airplanes during COVID-19. Noticeably, the three clusters were not significantly different in terms of their geographic locations, as indicated by the chi-square test.
Table 2. Profiling the Clusters with Demographic Factors
PREDICTIVE VALIDITY OF CLUSTERS – MULTINOMIAL LOGISTIC REGRESSION
Another purpose of this study was to determine which TPB constructs (attitude, subjective norms, and perceived behavioral control) most significantly influenced the formation of the three clusters identified in Section 4.1. The focus of this analysis was on identification of the key distinguishing factor that can be used to discriminate between air travelers in the Comfort First, Balanced, and Risk Avoider segments. To that end, multinomial logistic regression was performed to determine the ability of the three independent variables (attitude toward masks, subjective norms, and perceived behavioral control) to predict the dependent variable (classification of the respondents into the three clusters). While multinominal logistic regression does not require assumptions commonly required for many multivariate analysis techniques, it requires the absence of multicollinearity between independent variables. As shown in Table 4, all values of VIF were less than ten, indicating that no factor was redundant with the other factors in explaining the variance in cluster classification.
Table 3. Profiling the Clusters with Travel Experience and COVID-19 Factors
Table 4 shows the results of the multinomial logistic regression analysis. The estimated MLR model showed a significant improvement from the baseline model (χ2=1088.826, p<.001), providing support for cluster difference and predictive validity of the cluster solution identified in Section 4.1. The test of Pearson χ2 produced similar results, with a non- significant p value indicating good model fit to the data (1602.520, p=1.000). For the purpose of examining the impact of attitude, subjective norms, and PBC on cluster membership of the respondents, Comfort First was used as the reference group (or baseline category) against which the other two clusters were compared. Hence, the coefficients and odds ratio for Comfort First was represented by R in Table 4.
Table 4.
All the three factors were found to be significant, yet the magnitude of their impact varied substantially across the clusters. The comparison between the reference group of Comfort First (Cluster One) and Balanced Group (Cluster Two) indicated significance of all three factors. The regression coefficients associated with attitude and subjective norms were positive values (β=3.737 and β=0.817, respectively), suggesting that air travelers scored higher on attitude and subjective norms were more likely to belong to Balanced Group instead of Comfort First group. As PBC showed a negative coefficient (β=-.1669), individuals scored higher on perceived control were less likely to be in the Balanced Group than the Comfort First group. The likelihood of being in Clusters One and Cluster Two was given by the odds ratio. The odds ratio associated with attitude was 41.687, indicating that for one unit increase in the score of attitude toward masks, the odds of an air traveler been classified into Balanced Group rather than Comfort First group would increase by 40.687 units, holding subjective norms and perceived behavioral control constant.
Similarly, the odds ratio associated with subjective norms (2.264) indicated that for one unit increase in scores representing subjective norms, the odds of an air traveler being classified into Balanced Group would increase by 1.264 units. The negative coefficient for PBC indicated that for one unit increase in perceived control on mask-related issues, the odds of a traveler belonging to Balanced Group would decrease, holding the other two factors constant. Comparison between Cluster One (Comfort First) and Cluster Three (Risk Avoider) indicated that attitude was the only significant predictor with a positive regression coefficient (β=5.364) and a very large odds ratio of 213.665. This means that air travelers who scored higher in attitude toward masks were far more likely to be classified in Risk Avoider cluster instead of Comfort First cluster. Subjective norms and PBC were not significant when Clusters One and Three were compared. Clearly, attitude toward masks, compared to the other two TPB factors, was the key factor to predict air travelers’ classification into the three clusters.
Finally, the classification statistics in Table 4 quantify the accuracy of model prediction. Air travelers in Comfort First group (Cluster One) were correctly classified by the model at the rate of 88.7%, followed by 84% for Risk Avoider (Cluster Three), demonstrating the strong predictive power of the model regarding these two clusters. The model achieved a lower accuracy predicting the membership of Balanced Group (Cluster 2) (65.8%), likely due to the lack of clear characteristics of air travelers in this group (which was different from the other two clusters). Table 5 summarizes the unique characteristics of the three clusters.
Table 5. Passenger Characteristics in Three Clusters – Mask-Wearing on Airplanes in COVID-19
Discussion
The cluster analysis, using the three cluster variables (comfort, risk avoidance, and information-seeking), led to the formation of three passenger segments (Comfort First, Balanced Group, and Risk Avoider). Each segment yielded different results, indicating that the joint use of the cluster variables, instead of separate evaluations, can provide more meaningful insights into the group patterns of air travelers’ mask-wearing decisions onboard airplanes in COVID-19. The Comfort First segment assigned the highest value to comfort of mask-wearing and the lowest values to risk avoidance and information searching. This group is the smallest in size, likely representing air travelers who deny the seriousness of COVID-19, are not interested in seeking information about the pandemic, and are more concerned with the physical comfort of wearing a mask onboard an airplane. This finding is consistent with the literature that mentions a small but vocal group of individuals who are strongly opposed to mask use, often citing inconvenience and discomfort as the reasons for refusing to wear a mask.36,52 Previous studies have estimated that 15-20% of Canadian and American adults do not regularly wear masks during COVID-19,36 which is roughly equivalent in size to the Comfort First segment (almost 15% of the total respondents).
At the other end of the spectrum, the Risk Avoider segment was the largest group of travelers giving the highest values to risk avoidance and information-seeking and the lowest value to comfort of mask-wearing. This group likely represents air travelers who pay more attention to avoiding the risks of COVID-19, seek as much information about COVID-19 as possible to stay informed, and basically ignore the comfort of mask-wearing. Air travelers in this group seriously consider the risks of in-flight infection and are willing to use all available means to mitigate the risks, even when it could compromise their physical comfort. The identification of risk avoiders in COVID-19 and their mask-wearing behaviors are well documented in the literature, as numerous studies suggest a strong relationship between risk perception of COVID-19 and a willingness to use face masks.6,27,35 The Risk Avoider segment also demonstrated the greatest motivation to find information about COVID-19, when compared to the other two groups. For these travelers, their active search for information reinforced their risk perception of COVID-19, which enhanced their decision to wear a mask during flight.
Between the two extreme clusters was the Balanced Group segment, which has a balanced view about the importance of comfort, risk avoidance, and information-seeking when the three factors were evaluated simultaneously in the context of mask use onboard airplanes. This passenger segment is the second largest group in the study, accounting for one-third of the total respondents. Unlike the other two segments that exhibited vastly different views about the importance of the three factors, the air travelers in the Balanced Group prioritized risk avoidance of COVID-19, while recognizing the importance of information-seeking and comfort of mask-wearing. These findings show that, in the context of flying during COVID-19, many air travelers tend to balance comfort, risk avoidance, and information when they decide to wear or not wear a mask onboard an airplane. In other words, many air travelers prefer to avoid the risk of COVID-19 without sacrificing too much physical comfort during flight, a finding that has not been addressed previously in the literature.
The three segments exhibited different characteristics with regards to the thirteen variables that were not included in the clustering process, indicating that different demographic, travel, and mask-wearing patterns can be identified among air travelers onboard airplanes during COVID-19. The data shows that female travelers who had more education were more likely to be risk aversive about inflight infection, and there seemed to be a more balanced racial mix among these travelers. On the other hand, the predominantly White, male travelers and travelers with less education appeared to emphasize physical comfort rather than the risks of COVID-19 during flight. Moreover, the respondents from the three segments were not significantly different in terms of age, income, or geographic location. The finding of age is particularly surprising as the age factor has been frequently related to different mask-wearing perceptions and behaviors.53 The finding may be related to the examination of passenger clusters, rather than to individual travelers, when multiple COVID-19 factors are analyzed simultaneously. The three segments also demonstrated different travel behaviors as the Risk Avoider segment reduced travel frequency of air travel most extensively, compared to that of the other two segments. More than half of the Risk Avoider segment stopped flying at the start of the pandemic.
The Comfort First and the Risk Avoider segments showed the most differences in terms of their mask- wearing perceptions and behaviors, even though they had similar mask-wearing behaviors before COVID-19. Almost all of the members of these groups did not wear a mask before COVID-19 in crowded settings such as conferences and supermarkets, which agrees with the fact that face covering is not a tradition custom in the US. During COVID-19, however, almost all members of the Risk Avoider segment wear a mask, and nearly 80% of the Comfort First group do likewise. This difference is even more striking with regards to voluntary mask-wearing, where 95% of the Risk Avoider segment chose to wear a mask, and nearly the same percentage of the Comfort First segment chose not to do so. Thus, the different mask-wearing behaviors may be driven by different degrees of recognition and acceptance of the risks of COVID-19. Generally, air travelers who accept the seriousness of COVID-19 are more likely to wear a mask during flight, and the converse is also true. These findings are supported by previous studies that show a positive relationship between risk perception and willingness to wear a mask.6,35 The three segments also differed with regards to their perceived effectiveness of mask-wearing. The Risk Avoider group overwhelmingly believed that mask- wearing is an effective means to protect the wearer and others, while only a small number of those in the Comfort First group held the same view. This is consistent with numerous reports of the mixed views about mask-wearing during COVID-19.52
All three TPB constructs – attitude, subjective norms, and perceived behavioral control – can be used to predict the membership of the three segments, though their predictive power varies. Subjective norms and PBC can effectively predict the classification of air travelers in the Balanced Group, versus the two extreme segments (Comfort First and Risk Avoider). Most travelers who followed the expectations of their important ones for mask- wearing tended to be in the Balanced Group. Regarding PBC, the findings showed that air travelers with higher levels of perceived control in issues related to mask-wearing were likely classified in the Comfort First group or in the Risk Avoider group. Nevertheless, subjective norms and PBC were not significant in differentiating travelers in either of the two extreme segments (Comfort First and Risk Avoider). While previous studies have shown the importance of social norms and perceived control in mask use,7 the findings in this study show that normative and control influences may be less effective in predicting the passenger segments with extremely strong views about comfort and risk avoidance, with regards to mask-wearing on airplanes.
In this study, attitude toward mask-wearing was the only factor that could predict the classification of all three segments. The three segments demonstrated significant differences in attitude toward mask- wearing, with increasing positive attitude in order from the Comfort First group, to the Balanced Group, and to the Risk Avoider group. A large increase in favorable attitudes toward mask- wearing was seen when moving from the Comfort First group to the Balanced Group, though a more dramatic increase was seen when moving from the Comfort First group to Risk Avoider group. Thus, in the context of mask-wearing onboard airplanes during COVID-19, air travelers who prioritized risk avoidance (the Balanced Group and Risk Avoider group) held far more favorable attitudes toward mask-wearing than those who prioritized comfort (Comfort First group). These findings are consistent with the literature on the importance of attitude about mask use during COVID-19.25,36 This study adds to the literature by showing that attitude toward mask-wearing is significant when comparing passenger segments, and among the three TPB constructs, attitude is the key factor that distinguishes between the passenger segments in this study.
Conclusions
This study collected survey data about mask- wearing onboard airplanes during COVID-19, with the purpose of segmenting air travelers based on their views about the importance of comfort, risk avoidance, and information-seeking. The findings identify which TPB constructs – attitude, subjective norms, and perceived behavioral control – are the best for discriminating between the segments. Comfort, risk avoidance, and information-seeking were examined together as cluster variables to form the three clusters: 1) Comfort First, emphasizing comfort in mask-wearing onboard airplanes; 2) Risk Avoider, focusing mostly on avoiding risks of inflight transmission; and 3) Balanced Group, prioritizing risk avoidance of COVID-19, while also recognizing the importance of comfort and information-seeking about mask- wearing onboard airplanes. Overall, the three segments demonstrated different demographic, travel, and mask-use characteristics, supporting the validity of the cluster results. Of the three TPB constructs, attitude was the only one that could significantly predict membership in all of the passenger segments.
The findings of this study are important in several ways. To the best of our knowledge, this study is the first to examine mask use of air travelers from the perspective of passenger segments, contributing to an understanding of mask use during a global pandemic. At a practical level, this study can inform airlines and aviation policy-makers in developing health and safety strategies. By understanding different passenger groups and how they evaluate the importance of comfort, risk avoidance, and information-seeking with regards to mask-wearing onboard airplanes, airlines may be better able to develop strategies to help the industry recover from the pandemic. In particular, the finding of this study that the largest passenger segment assigned the highest level of importance to risk avoidance onboard airplanes, may help guide airlines to develop strategies for the long-term distribution of free masks. In addition, given the importance of attitude in distinguishing between passenger segments with regards to mask-wearing onboard airplanes, more effort should be made to promote positive attitudes toward mask use in society.
Role of the funding source
This research was supported by the Center for Advanced Transportation Mobility (CATM), USDOT grant # 69A3551747125.
Conflict of interest statement
There is no conflict of interest
References
1. Hotle S, Mumbower S. The Impact of COVID-19 on Domestic U.S. Air Travel Operations and Commercial Airport Service. Transportation Research Interdisciplinary Perspectives. 2020;9:100277. https://doi.org/10.1016/j.trip.2020.100277
2. Kleitman S, Fullerton DJ, Zhang LM, Blanchard MD, Lee J, Stankov L, Thompson V. To comply or not comply? A latent profile analysis of behaviours and attitudes during the COVID-19 pandemic. PloS One. 2021;16(7):e0255268. https://doi.org/10.1371/journal.pone.02552 68
3. Abboah-Offei M, Salifu Y, Adewale B, Bayuo J, Ofosu-Poku R, Opare-Lokko EBA. A rapid review of the use of face mask in preventing the spread of COVID-19. International Journal of Nursing Studies Advances. 2021;3(3):100013. https://doi.org/10.1016/j.ijnsa.2020.100013
4. Tao Z, Dong J, Culleton R. The use of facemasks may not lead to an increase in hand–face contact. Transbound Emerg Dis. 2020;67(6), 3038-3040. https://doi.org/10.1111/tbed.13698
5. Wang Y, Tian H, Zhang L, et al. Reduction of secondary transmission of SARS-CoV-2 in households by face mask use, disinfection and social distancing: a cohort study in Beijing, China. BMJ Global Health. 2020;5(5):e002794. https://doi.org/10.1136/bmjgh-2020- 002794
6. Irfan M, Akhtar N, Ahmad M, et al. Assessing Public Willingness to Wear Face Masks during the COVID-19 Pandemic: Fresh Insights from the Theory of Planned Behavior. International Journal of Environmental Research and Public Health. 2021;18(9):4577. https://doi.org/10.3390/ijerph18094577
7. Sun Y, Qin B, Hu Z, et al. Predicting mask- wearing behavior intention among international students during COVID-19 based on the theory of planned behavior. Annals of Palliative Medicine. 2021;10(2):55-55. https://doi.org/10.21037/apm-20-2242
8. Hair, JF, Black, WC, Babin, BJ, Anderson, RE. Multivariate data analysis, New Jersey: Cengage; 2019.
9. Chen HT, Chao CC. Airline choice by passengers from Taiwan and China: A case study of outgoing passengers from Kaohsiung International Airport. Journal of Air Transport Management. 2015;49:53-63. https://doi.org/10.1016/j.jairtraman.2015.0 8.002
10. Fu X, Juan Z. Empirical analysis and comparisons about time-allocation patterns across segments based on mode-specifi preferences, Transportation. 2016;43:37-51. https://doi.org/10.1007/s11116-014-9561- 2
11. Kuljanin JM, Kalić. Exploring characteristics of passengers using traditional and low-cost airlines: A case study of Belgrade Airport. JATM. 2015;46:12 18. http://doi.org/10.1016/j.jairtraman.2015.03.009
12. Wartelle A, Mourad-Chehade F, Yalaoui F, Chrusciel J, Laplanche D, Sanchez S. Effect of the COVID-19 pandemic lockdown on non- COVID-19 emergency department visits in Eastern France: Reduced risk or avoidance behavior? Public Health in Practice. 2021;2:100109. https://doi.org/10.1016/j.puhip.2021.10010 9
13. Dzimińska P, Drzewiecki S, Ruman M, Kosek K, Mikołajewski K, Licznar P. The Use of Cluster Analysis to Evaluate the Impact of COVID-19 Pandemic on Daily Water Demand Patterns. Sustainability. 2021;13(11):5772. https://doi.org/10.3390/su13115772
14. García S, Parejo A, Personal E, Ignacio Guerrero J, Biscarri F, León C. A retrospective analysis of the impact of the COVID-19 restrictions on energy consumption at a disaggregated level. Applied Energy. 2021;287:116547. https://doi.org/10.1016/j.apenergy.2021.11 6547
15. Hartono A, Ishak A, Abdurrahman A, et al. COVID-19 Pandemic and Adaptive Shopping Patterns: An Insight from Indonesian Consumers. Global Business Review. Published online June 18, 2021:097215092110135. https://doi.org/10.1177/09721509211013 512
16. Theriot A, Urrutia-Alvarez N, McKinley EM. An Analysis of Pandemic Panic Buying Motivators among Undergraduate College Students Using Mind Genomics Cognitive Science. Psychology. 2021;12(09):1457-1471. https://doi.org/10.4236/psych.2021.129092
17. Ahmed MS, Aurpa TT, Anwar MM. Detecting sentiment dynamics and clusters of Twitter users for trending topics in COVID-19 pandemic. Cherifi H, ed. PLOS ONE. 2021;16(8):e0253300. https://doi.org/10.1371/journal.pone.02533 00
18. Enders AM, Uscinski J, Klofstad C, Stoler J. On the relationship between conspiracy theory beliefs, misinformation, and vaccine hesitancy. Jonason PK, ed. PLOS ONE. 2022;17(10):e0276082. https://doi.org/10.1371/journal.pone.02760 82
19. Lang R, Benham JL, Atabati O, et al. Attitudes, behaviours and barriers to public health measures for COVID-19: a survey to inform public health messaging. BMC Public Health. 2021;21(1). https://doi.org/10.1186/s12889-021- 10790-0
20. Sheng X, Ketron SC, Wan Y. Identifying consumer segments based on COVID ‐19 pandemic perceptions and responses. Journal of Consumer Affairs. 2021;56(1). https://doi.org/10.1111/joca.12413
21. Kopsidas A, Milioti C, Kepaptsoglou K, Vlachogianni EI. How did the COVID-19 pandemic impact traveler behavior toward public transport? The case of Athens, Greece. Transportation Letters. Published online March 23, 2021:1-9. https://doi.org/10.1080/19427867.2021.19 01029
22. Budd T, Suau-Sanchez P, Halpern N, Mwesiumo D, Bråthen S. An assessment of air passenger confidence a year into the COVID-19 crisis: A segmentation analysis of passengers in Norway. Journal of Transport Geography. 2021;96:103204. https://doi.org/10.1016/j.jtrangeo.2021.103 204
23. Gray L, MacDonald C, Tassell-Matamua N, et al. Wearing one for the team: views and attitudes to face covering in New Zealand/Aotearoa during COVID-19 Alert Level 4 lockdown. J Prim Health Care. 2020;12(3):199. https://doi.org/10.1071/hc20089
24. Howard MC. Gender, face mask perceptions, and face mask wearing: Are men being dangerous during the COVID-19 pandemic? Personality and Individual Differences. 2021;170:110417. https://doi.org/10.1016/j.paid.2020.11041 7
25. Cheok GJW, Gatot C, Sim CHS, et al. Appropriate attitude promotes mask wearing in spite of a significant experience of varying discomfort. Infection, Disease & Health. 2021;26(2):145-151. https://doi.org/10.1016/j.idh.2021.01.002
26. Lorian CN, Grisham JR. Clinical implications of risk aversion: An online study of risk-avoidance and treatment utilization in pathological anxiety. Journal of Anxiety Disorders. 2011;25(6):840-848. https://doi.org/10.1016/j.janxdis.2011.04.0 08
27. Bruine de Bruin WB, Bennett D. Relationships Between Initial COVID-19 Risk Perceptions and Protective Health Behaviors: A National Survey. American Journal of Preventive Medicine. Published online May 2020. https://doi.org/10.1016/j.amepre.2020.05.0 01
28. Hopfer S, Fields EJ, Lu Y, et al. The social amplification and attenuation of COVID-19 risk perception shaping mask wearing behavior: A longitudinal twitter analysis. Gesser-Edelsburg A, ed. PLOS ONE. 2021;16(9):e0257428. https://doi.org/10.1371/journal.pone.02574 28
29. Bento AI, Nguyen T, Wing C, Lozano-Rojas F, Ahn YY, Simon K. Evidence from internet search data shows information-seeking responses to news of local COVID-19 cases. Proceedings of the National Academy of Sciences. 2020;117(21):11220-11222. https://doi.org/10.1073/pnas.2005335117
30. Soroya SH, Farooq A, Mahmood K, Isoaho J, Zara S. From information seeking to information avoidance: Understanding the health information behavior during a global health crisis. Information Processing & Management. 2021;58(2):102440. https://doi.org/10.1016/j.ipm.2020.102440
31. Kim YJ, Cho J, Kang SW. Study on the Relationship between Leisure Activity Participation and Wearing a Mask among Koreans during COVID-19 Crisis: Using TPB Model. International Journal of Environmental Research and Public Health. 2020;17(20):7674. https://doi.org/10.3390/ijerph17207674
32. Buaphiban T, Truong D. Evaluation of passengers’ buying behaviors toward low cost carriers in Southeast Asia. Journal of Air Transport Management. 2017;59:124-133. https://doi.org/10.1016/j.jairtraman.2016.1 2.003
33. Hsiao CH, Yang C. Predicting the travel intention to take High Speed Rail among college students. Transportation Research Part F: Traffic Psychology and Behaviour. 2010;13(4):277-287. https://doi.org/10.1016/j.trf.2010.04.011
34. Pan JY, Truong D. Passengers’ intentions to use low-cost carriers: An extended theory of planned behavior model. Journal of Air Transport Management. 2018;69:38-48. https://doi.org/10.1016/j.jairtraman.2018.0 1.006
35. Si H, Shen L, Liu W, Wu G. Uncovering people’s mask-saving intentions and behaviors in the post-COVID-19 period: Evidence from China. Sustainable Cities and Society. 2021;65:102626. https://doi.org/10.1016/j.scs.2020.102626
36. Taylor S, Asmundson GJG. Negative Attitudes about Facemasks during the COVID-19 pandemic: the Dual Importance of Perceived Ineffectiveness and Psychological Reactance. Capraro V, ed. PLOS ONE. 2021;16(2):e0246317. https://doi.org/10.1371/journal.pone.02463 17
37. Zhang B, Li Z, Jiang L. The Intentions to Wear Face Masks and the Differences in Preventive Behaviors between Urban and Rural Areas during COVID-19: An Analysis Based on the Technology Acceptance Model. International Journal of Environmental Research and Public Health. 2021;18(19):9988. https://doi.org/10.3390/ijerph18199988
38. Young SD, Goldstein NJ. Applying social norms interventions to increase adherence to COVID- 19 prevention and control guidelines. Preventive Medicine. 2021;145:106424. https://doi.org/10.1016/j.ypmed.2021.1064 24
39. Barceló J, Sheen GCH. Voluntary adoption of social welfare-enhancing behavior: Mask- wearing in Spain during the COVID-19 outbreak. Shaman J, ed. PLOS ONE. 2020;15(12):e0242764. https://doi.org/10.1371/journal.pone.02427 64
40. Aschwanden D, Strickhouser JE, Sesker AA, et al. Preventive Behaviors During the COVID-19 Pandemic: Associations With Perceived Behavioral Control, Attitudes, and Subjective Norm. Frontiers in Public Health. 2021;9. https://doi.org/10.3389/fpubh.2021.66283 5
41. Bao Y, Zhou KZ, Su C. Face consciousness and risk aversion: Do they affect consumer decision- making? Psychology and Marketing. 2003;20(8):733-755. https://doi.org/10.1002/mar.10094
42. DuBenske LL, Burke Beckjord E, Hawkins RP, Gustafson DH. Psychometric Evaluation of the Health Information Orientation Scale. Journal of Health Psychology. 2009;14(6):721-730. https://doi.org/10.1177/13591053093388 92
43. Harvey J, Thorpe N, Caygill M, Namdeo A. Public attitudes to and perceptions of high speed rail in the UK. Transport Policy. 2014;36:70-78. https://doi.org/10.1016/j.tranpol.2014.07.0 08
44. Jing P, Juan Z. (2013). The Theory of Planned Behavior: The role of descriptive norms and habit in the prediction of inter-city travel mode choice, Journal of Convergence Information Technology. 2013;8(10):211-219.
45. Jing P, Juan Z, Gao LJ. Application of the Expanded Theory of Planned Behavior in Intercity Travel Behavior. Discrete Dynamics in Nature and Society. 2014;2014:1-10. https://doi.org/10.1155/2014/308674
46. Meertens RM, Lion R. Measuring an Individual’s Tendency to Take Risks: The Risk Propensity Scale. Journal of Applied Social Psychology. 2008;38(6):1506-1520. https://doi.org/10.1111/j.1559- 1816.2008.00357.x
47. Oborne DJ, Clarke MJ. Questionnaire surveys of passenger comfort. Applied Ergonomics. 1975;6(2):97-103. https://doi.org/10.1016/0003- 6870(75)90302-6
48. Park HS, Klein KA, Smith S, Martell D. Separating Subjective Norms, University Descriptive and Injunctive Norms, and U.S. Descriptive and Injunctive Norms for Drinking Behavior Intentions. Health Communication. 2009;24(8):746-751. https://doi.org/10.1080/10410230903265 912
49. Raju PS. Optimum Stimulation Level: Its Relationship to Personality, Demographics, and Exploratory Behavior. Journal of Consumer Research. 1980;7(3):272. https://doi.org/10.1086/208815
50. Taylor S, Todd PA. Understanding Information Technology Usage: A Test of Competing Models. Information Systems Research. 1995;6(2):144- 176. https://doi.org/10.1287/isre.6.2.144
51. Yang ZJ, Kahlor L. What, Me Worry? The Role of Affect in Information Seeking and Avoidance. Science Communication. 2012;35(2):189-212. https://doi.org/10.1177/10755470124418 73
52. Scheid JL, Lupien SP, Ford GS, West SL. Commentary: Physiological and Psychological Impact of Face Mask Usage during the COVID- 19 Pandemic. International Journal of Environmental Research and Public Health. 2020;17(18):6655. https://doi.org/10.3390/ijerph17186655
53. Haischer MH, Beilfuss R, Hart MR, et al. Who is wearing a mask? Gender-, age-, and location- related differences during the COVID-19 pandemic. Kotozaki Y, ed. PLOS ONE. 2020;15(10):e0240785. https://doi.org/10.1371/journal.pone.02407 85
Author Area
Have an article to submit?
Submission Guidelines
Submit a manuscript
Become a member