Home > Medical Research Archives > Issue 149 > Atherosclerosis Detection using Autoencoders Applied to MR Images
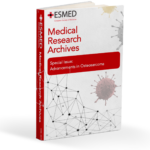
Published in the Medical Research Archives
Sep 2023 Issue
Atherosclerosis Detection using Autoencoders Applied to MR Images
Published on Sep 28, 2023
DOI
Abstract
The early detection of atherosclerosis has been the interest of researchers in order to prevent diseases progression. And, most of cases diagnosed with atherosclerosis in late stages resulted of subjective ways of diagnosis in the healthcare sector.
Consequently, in order to help cardiologists diagnose atherosclerosis in early stages with an objective, fast and accurate way, we are proposing a machine learning model based on autoencoders that enables atherosclerosis detection from MRI images of murine subjects.
This novel way of automated system consists of applying various image processing techniques on the input MRI images. Then, after training, testing and validation it will be capable of classifying the images as atherosclerotic or not based on a specific threshold for the reconstruction error calculated from the autoencoders' output.
An autoencoder is a feed-forward neural network that has its input neurons equal to the output neuron. The classification works by comparing the reconstructed images to the original input images and evaluating loss between them. Since the autoencoder is trained on healthy images, reconstruction error of the healthy images would be low while that of atherosclerotic subjects would be higher. By setting a threshold for the loss, we can classify the images as healthy or atherosclerotic.
The pre-processing of these images was made using a Block Matching 3D (BM3D) filter to remove the noise in the images prior to application of a Contrast Limited Adaptive Histogram Equalization (CLAHE) to enhance the contrast. The next step included introducing the dataset into the autoencoder to start training on the healthy images, after increasing the images number with augmentation.
The results showed a reconstruction loss of 0.018 while using the stacked architecture of the autoencoder and 0.0366 when using the convolutional autoencoder architecture.
Author info
Author Area
Have an article to submit?
Submission Guidelines
Submit a manuscript
Become a member