Home > Medical Research Archives > Issue 149 > A Machine Learning Approach to Classify Exercise Limitation Severity Using Cardiopulmonary Exercise Testing - Development and Validation
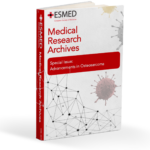
Published in the Medical Research Archives
Nov 2023 Issue
A Machine Learning Approach to Classify Exercise Limitation Severity Using Cardiopulmonary Exercise Testing - Development and Validation
Published on Nov 29, 2023
DOI
Abstract
Background: There is no agreement on the best strategy for interpreting/ analyzing cardiopulmonary exercise test (CPET) results.
Aims: This study aims to evaluate the feasibility of using computer-aided algorithms to evaluate CPET data to identify the exercise limitation/ intolerance level.
Methods: This study used 206 retrospective CPET files from the Pulmonary Institute and the Cardiology Rehabilitation Center at the Sheba Medical Center and 50 from the exercise physiology laboratory at the Washington-Hill College, both in Israel. Eighty patients with confirmed primary cardiovascular-related exercise limitation, seventy-six with ventilatory-related exercise limitation, and fifty healthy (none or very mild exercise restraints) subjects comprised the pool of examined CPET data. Support Vector Machine (SVM) Learning was performed on 150 (50 in each group) of the 206 CPETs, while model validation was performed on the remaining 56 CPET files. By applying the K-means clustering method, distribution analysis was used to compare the SVM interpretive module's performance to that of senior cardiologists, pulmonologists, and expert exercise physiologists.
Results: Overall, the proposed interpretive model has a predictive power of between 78% and 100%, as shown by its ability to correctly classify the degree of exercise limitation.
Conclusions: The proposed machine-learning CPET interpretive module was highly sensitive and specific in identifying patients with mild, moderate, or severe cardiovascular- or ventilatory-related exercise limitations/ intolerance. Comparable modules may be applied to additional (kinds of exercise limitations) and larger populations, making this tool powerful and clinically applicable.
Author info
Author Area
Have an article to submit?
Submission Guidelines
Submit a manuscript
Become a member